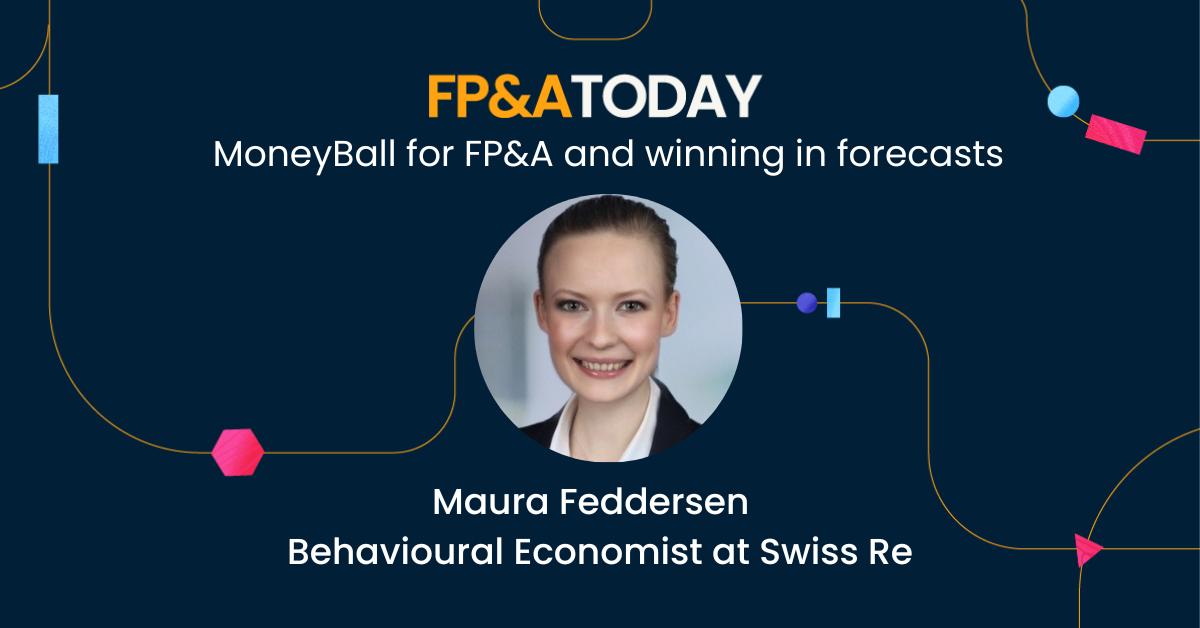
Maura Feddersen, behavioral research manager at Swiss Re, is an economist who previously worked in the economics team of two of the Big Four Accountancy firms: PwC and KPMG.
She got switched on to behavioral economics as “I always felt there was something a bit missing in economics. [Economics] assumes that people act quite rationally…and that was just not the case. Behavioral economics brings together the economics, as well as insights from psychology, to try to assess how humans really make decisions”.
This fascinating episode with Maura, uncovers the lessons that FP&A teams can learn from behavioral economics to improve forecasting and the power of “knowing what knowing what you don’t know.”
This episode includes
- New research revealing that investors predictions are only slightly more accurate than a chimp
- How new forecasting methods improved accuracy at Swiss Re by 5%
- The cognitive biases we need to be aware of that undermine accuracy (such as the dangers of groupthink)
- Meryl Streep’s Oscar nominations and your forecast confidence levels tested
- How to try and manage cognitive bias
- The true economic impact of uncertainty in the global economy and in your business
- The opportunities and limits of AI forecasting
- Using RIO (Rational Impartial Observers)
- The biggest failure in her career
- Favorite Excel function
- Her biggest advice for someone starting out to get really good at forecasting
Further reading
Actuaries magazine: So You Think You Can Underwrite [Maura Fedderson]
Insurers, take heed of these 3 common forecasting fallacies [by Maura Feddersen]
Recommended books
Superforecasting: The Art and Science of Prediction Paperback – Philip Tetlock, Dan Gardner
Nassim Taleb, The Black Swan, the Impact of the Highly Improbable
Adam Grant, Think Again, The Power of Knowing what you Don’t Know
Daniel Kahneman, Olivier Sibony, Cass R. Sunstein, Noise: A Flaw in Human Judgment
Read the full transcript and blog below
Follow Maura Fedderson on LinkedIn
Follow Paul Barnhurst on LinkedIn
FP&A Today is brought to you by Datarails.
Datarails is the financial planning and analysis platform that automates data consolidation, reporting and planning, while enabling finance teams to continue using their own Excel spreadsheets and financial models.
Get in touch at www.datarails.com
For AFP FP&A Continuing Education credit please complete the course via the Earmark Ap, must pass the quiz with 80% accuracy and send the completed certificate to pbarnhust@thefpandaguy.com for issuance of 1 hour of credit toward your AFP FP&A Certification.
Paul Barnhurst:
Hello everyone. Welcome to FP&A Today I am your host, Paul Barners, aka the FP&A Guy, and you are listening to FP&A Today. FP&A Today is brought to you by Data Rails, the financial planning and analysis platform for Excel users. Every week we welcome a leader from the world of financial planning and analysis and discuss some of the biggest stories and challenges in the world of fp and a. We’ll provide you with actual advice about financial planning and analysis. This is going to be your go-to resource for everything FP&A. I’m thrilled to welcome today’s guest on the show, Maura Feddersen. Mara, welcome to the show.
Maura Feddersen:
Thanks so much, Paul for having me.
Paul Barnhurst:
Yeah, no, really excited you could join us. So this is going to be a really exciting episode. Mara comes to us from London. She earned her bachelor’s in master’s degree in economics. She currently works for Swiss Re, not Credit Suisse, as I accidentally said, as I was chatting with her before the interview as a behavioral economist and she previously worked for both PWC and KP&G South Africa as an economist. So Maura, maybe you could start by just kind of telling us a little bit about yourself and your background.
Maura Feddersen:
Happy to. So I’m an economist by background and I used to work in the economics teams, as you said, of two of the big four accounting firms. And it was a really interesting time to work there because it was a transition at the time to IFRS 9, the new accounting standard for many banks. And it was also a transition from backward looking to forward-looking financial reporting. And at that time there was a big focus on how is it possible to audit the economics components of these forward looking financial views. So that was a very interesting challenge to look at. In addition, I’d always had a really strong interest in psychology and what really drives human behavior. And so at the same time as I was doing that, I was also studying more about that topic through the lens of behavioral economics. And at one point I had an opportunity to pivot more in that direction. So what I do currently is combine a little bit those two parts of my background. I help underwriters, actuaries, economists forecast more accurately by better navigating some of the cognitive biases that might undermine their expert judgment. So you can think of it a little bit as sort of a Moneyball coach for actuaries.
Paul Barnhurst:
I like that. I liked like the Moneyball analogy. That’s a good way to put it. And I know a lot of people love that book and will relate to it. So thank you for that analogy there. I do like that. So you know mentioned you started kind of economics started getting into the psychology side and the behavioral. So what made you decide to become a behavioral economist? What is it that you know like about that for kind of day to day?
Maura Feddersen:
Yeah, there was something I thought was a little bit missing in economics. It really assumes that people act quite rationally, are able to process a lot of information and make decisions that really align with what they’re looking for in the short term but also longer term. And my feeling was that that was just not the case and I found some of the answers, or at least I thought I found some of the answers by looking more into the space of psychology. And behavioral economics really brings together the economics, the economic way of thinking as well as insights from psychology to try to assess how humans really make decisions. And the other thing that I think behavioral economics brings is a very evidence based approach. A lot of focus on testing what works on the ground, what doesn’t work, and building on that iteratively.
Paul Barnhurst:
I like how you explain that and I think it’s very interesting how you mentioned right economics. I think everybody remembers their economics courses. I know I do. And very much the assumption that everybody via irrational behavior that you’re going to maximize your benefit. And so often that’s not true, like sunk cost. How often do we make a decision just because, well I’ve spent the money and so I’m going whether I want to go or not because I can’t let that money go. Things like that. So I think psychology brings a nice balance to the economic side and the reality is many of us don’t behave rationally. I know I haven’t and I’ve definitely seen plenty of my lifetime we’re watching people and going that definitely wasn’t rational by any stretch of the imagination. Right. So why do you think that is? Why do you think most people don’t behave rationally that it’s so important to understand the psychology side of things?
Maura Feddersen:
I think it’s exactly that. I think there’s a lot that’s going on the partially subconsciously that is simply not explained by economic models. And already these are highly complex trying to account for utility changes in prices. These are already sophisticated mathematical models, but then we are not only driven by those incentives that you can measure in that way. So I think behavioral economics tries to shed a little bit more light on that. And it’s true, it’s exactly those examples that you share that really stand out. For example, buying something on Black Friday that turns out you didn’t really need but you saw everyone else rushing. So we feel it ourselves in our decision-making and that’s definitely also what intrigued me about behavioral economics.
Paul Barnhurst:
I love the example of Black Friday because how often if somebody came back with something but it was a really good deal. Well, was it if you don’t need it and you’re never going to use it but the brain, that kind of how marketing works, there’s a lot of things we do that aren’t rational. And the second thing I’ve always kind of joked when it comes to economics, right, ask four economists get five opinions because it’s complex. People are like, well the market’s going to do this and the economy’s going to do that. And I’m always like, when they’re a hundred percent sure I kind of discount it like okay, I get that you think this may be what happens, but there’s so many variables. It seems to me that it can be often very challenging. Has that kind of been your experience to try to predict where things are going? Do you find it pretty hard with all the different variables in economics?
Maura Feddersen:
I think there’s a lot of research out there that suggest paundits are often wrong. I think of there’s some very interesting research by Phillip Tetlock and his co-authors where he found that investors are typically on average not that much more correct than an average chimp, which is quite a harsh thing to say. But it sort of shifts I think the lens a little bit to allow us to realize it is actually very difficult to predict. There is that often used statement, it’s how to make predictions especially about the future and it’s been ascribed to many, many people. But any economist, I think that speaks with a lot of confidence and essentially says this will definitely happen. I think it’s worth taking with a pinch of salt. But actually I think a lot of economists tend to speak with a lot of caveats and so sometimes it is actually quite hard to extract what is the key message here to take away. So I think they do are on the side of caution typically.
Paul Barnhurst:
And I would agree with that. I think a lot of times you say, well this is what we think could happen assuming this happens and based on historical trends and all the caveats, just like when you read research, it’s like well here’s our conclusion but we only look at these five things and these other 10 things could completely change what we said is happening here. That type of, so that makes a lot of sense. Can you maybe talk a little bit about how you use behavioral and decision science, how behavioral and decision science can help improve forecasting? Why are those two things so important to forecasting?
Maura Feddersen:
So essentially there are a number of cognitive biases that can undermine the way in which we perceive information, process it and develop our expert judgment as well as the way in which we can learn from our forecast and our accuracy. And so we need an understanding of what these biases are, how they can undermine our accuracy in order to improve. And there are a bunch of tools that we can apply for, especially for key predictions to allow us to better navigate them. So for example, typically we are overconfident. So for example, if I give you a little bit of a quiz and I say to you, what is a range that you are very sure contains the correct number of academy award nominations that Meryl Streep has received, where you essentially 90% sure that your range from low to high contains that correct answer, what essentially is that range that you would come up with?
Paul Barnhurst:
And I’ll say one to 20.
Oh, that’s pretty good. That’s very good. I
Knew I had to go big just because I read your article.
Maura Feddersen:
Very good. So the answer here is 21, so you just missed it. Yes. Stop. Yeah,
Paul Barnhurst:
I almost said higher, but my mistake.
Maura Feddersen:
Yeah, I think you are trying to obviously find a range that is not super large. You’re trying to fit it. And so this is a very typical response. I think people are very surprised if you add a few more of these types of questions and you say to people, you’re aiming for about 90% confidence, they tend to come up with ranges that are too narrow so only four or five in 10 of their ranges are wide enough to contain the answer. And so what that suggests is a level of overconfidence. I’m essentially missing some things on both ends of the spectrum on the lower end maybe there’s a downside scenario that I’m not properly thinking about on the upper end. Maybe there’s an upper case that I’m not thinking about. So it’s suggest there’s a blind spot and that’s one of the biases that we essentially would want to try to alleviate. So yeah, it’s about accounting for the uncertainty. For example, for me, I don’t know a lot about actors or movies, so I would tend to ideally make the range quite wide. If it’s a forecast about inflation and I have been studying up on this topic and been following what the Federal Reserve Bank says or the Bank of England, maybe I can make my range a little bit more narrow. So just playing with that is one of the things then that you can start to think about when you want to address this cognitive bias in forecasting.
Paul Barnhurst:
I really like the example you shared and how most people get it wrong. And I think two examples that come to mind for me, and this isn’t necessarily forecasting but it speaks to the overconfidence as a cognitive bias. If you ask the average driver if they’re above average, most results are like 70, 80% of all drivers are above average. Well only 50. Yeah, you’re going to have half above and half below, hence the kind of average. But all of us think we’re above average. It’s also similar when you ask people usually, hey, are you above average in Excel using Microsoft Excel? And everybody’s, oh yeah, I’m above average. And then you walk through and see what they can do and you’re like, okay, well I don’t know that your confidence and reality are aligned. And I think that’s a common mistake we can make. So I really, I like the example there. Obviously you can widen the range, but what are some of the things we can do to overcome cognitive bias when we’re forecasting manage that?
Maura Feddersen:
I think managing is a really good word. I think it’s very difficult to overcome our nature. There is a benefit in thinking with these mental shortcuts and it’s served us well to be able to respond quickly. But absolutely they are, when you are coming into the domain of expert judgment and forecasting, it really makes sense not to be misled by these cognitive biases. So thinking in ranges is actually one of the things you can do. Just being conscious that you might need to make your range of what you think is feasible even wider than you thought you needed to. And then you can take it one step further and say, actually I want to think through what could be happening on either side of the base case in the form of a scenario. So if for example, I imagine things to go well, sales might go up, I might be able to raise prices, so that could be a good scenario. What’s the likelihood in my mind that this could happen? So that’s the upper case. What might be happening on the lower case side, maybe inflation goes up, maybe interest rates go up, what might happen there? What’s the likelihood and what might that mean for the financial parameter? Maybe that’s under consideration. And do I need to take any actions on the back of this? Am I concerned about liquidity for example? So thinking in scenarios is a really powerful next step as well.
Paul Barnhurst:
And I really like when I used the word manage. I heard when we had an FP and a expert on and she started to refer to it, she goes, I don’t call it scenario planning, I call it scenario management, you’re managing scenarios. It’s more than just a plan just to, hey, throw a couple numbers together. But the other thing I was going to say kind of a little bit back to cognitive bias, but imagine it all that I thought was really interesting is I have a friend who put together an article or so did a bunch of research and would go speak to companies about inflation and how to manage it. And in his research what he found is someone had done a study where they’d interviewed a bunch of CEOs and asked, Hey, if you rise, if raise price x amount, how many customers are you going to lose?
Right? What’s going to be the impact your customers? And on average these executive leaders doubled what the actual impact was going to be in this case because it was downside, right? They were thinking it’s going to be way worse. We can’t raise prices, everybody leaves. And the reality was the losses were almost always substantially less than what they forecasted. And I just thought that was a really interesting example for me of the kind of that bias and how sometimes we awfulize things think sometimes you go to the worst case, not in all things, but in certain areas you may do that, especially when you’re nervous of what the impact could be on raising prices. And so I thought that was a really interesting study to just show how wrong we can be sometimes with our assumptions
Maura Feddersen:
We could be wrong in both directions so we could be over optimistic or too pessimistic. And that’s also what I’ve been finding that often people are thinking they have been maybe too conservative in their costing or pricing or too optimistic, but they certainly don’t know exactly where they’ve done. They haven’t received any feedback to know whether they are well calibrated or not. So one of the things most powerful things you can do is just to try close that feedback loop and compare what was the expectation versus what happened and therefore was I right? Was I wrong? What was my reasoning? So allowing for that retrospective is a very powerful tool here.
Paul Barnhurst:
I agree you can learn a lot from what we call postmortem, right? Going back after the fact and going back and looking at, okay, why did we miss or why were we right? What assumptions made sense? What assumptions should have we adjusted? And so that you have an understanding for next time because the reality is rarely do we forecast something once and then we’re done. Especially in planning and budgeting. Every year you’re building a forecast and the more knowledge you learn, the more you learn about the industry, the environment, the factors, the better you can forecast. I’m curious in scenario management and just kind of your thoughts, have you used Monkey Carlos simulation or any kind of probabilities or what role do you think that plays in scenario management? Right, some kind of statistical modeling.
Maura Feddersen:
I would say that ideally you are bringing together the statistical models like Monte Carlo and you are also infusing a level of expertise, expert judgment. And ultimately what the research has shown is that when you bring those two together in a meaningful way, that’s when you can most enhance forecasting accuracy. So they’re really, it’s not an either or I would say, but rather they’re really powerful allies here.
Paul Barnhurst:
And how have you found it works best to bring those together? Do you find a lot of people, maybe the underwriters push back or that it’s hard cause it may not always be the same people. I would imagine sometimes you’re coming in and looking at things and taking a different approach than what they’re using. So how do you manage that and help people see the benefit of both sides?
Maura Feddersen:
So ultimately it’s about, from my experience, essentially saying to underwriters, actuaries, you have your process, some of your expert judgment is currently implicit, let’s make it explicit. And in that way we can make it more systematic, more repeatable. It’s documented, you can come back to it and learn from it. And I think typically that helps. I also think that what distinguishes, for example a more junior underwriter actually from a more senior one is they have developed an understanding, they have a lot of experience that they can draw from. And so essentially it’s about valuing that because it offers a lot in terms of additional forecasting accuracy. So we want to reap the benefits of that expertise. And the way to do that is by applying some of these methods like thinking in ranges, scenarios, et cetera. So I found that this is a helpful way of looking at it.
Paul Barnhurst:
That makes sense to me. I really like the part where you said, right, taking what they know implicitly and making it explicit. When I think about that, I think of a lot of times in FP&A forecasting, a lot of it’s driver based. It’s working with the experts to really understand what are those key drivers. They may implicitly know, hey, to forecast this, I know if you tell me we’re going to do this much in sales, I need this many people. It’s like, well how do you know that? What’s the driver that tells you that? Walk me through the process. So then you said you can document it and put it in a model. So next time it’s like, okay, well I’m growing this much, I need roughly this much. And ask ’em, Hey, does that make sense? And what’s kind of the range to that? So I think that’s a really good point about helping get out what they have implicit and make it explicit in the models because that improves the accuracy and it also helps you so you don’t lose the knowledge if something happened, right? They walk out the door tomorrow and it’s like, well nobody understands what Joe was doing. I think we’ve all seen that happen before. I know I have.
Maura Feddersen:
So absolutely. And even the idea of, again, thinking and ranges, if I say I have a really wide range that already allows much more of a fertile ground for further discussion, you’re essentially saying, okay, your range is this, my range is that. Your range is a lot narrower and mine is wider. Why is that the case? So if I said though I have a point estimate of a forecast, I think inflation is going to be X. That sort of stops the conversation right there. And if anyone were to discuss it with me, I might say, well are you doubting my expert judgment and there’s a little bit of confirmation bias there and I need to defend my judgment towards myself and others. And so it, it’s a really useful to try keep that and make that uncertainty explicit and keep talking about it while you’re in the early stages of thinking about what’s next.
Speaker 1:
[Datarails ad]
I really like that point about the uncertainty. And kind of along those lines, I know you wrote a piece for The Actuary in which you talked about the cost of uncertainty to the global economy and I think you had mentioned you had estimated to reduce global growth uncertainty by 35 basis points roughly the equivalent to size of the Finish economy. So can you talk a little bit about how uncertainty has such a big impact on the economy?
Maura Feddersen:
So if you think of firms and their investment decisions, for example, if you have a lot of uncertainty about what’s going to happen in the economy, what will consumers be looking for, what prices are they willing to pay, what might be happening with regulation? All of that might essentially cause, if it’s high uncertainty, affirm to delay investing, especially in those types of tools or in the infrastructure where the return may take a few years to materialize. And so essentially that is how you end up undermining economic growth. You’re essentially not having the investment in the fixed tools, machinery, roads, bridges, but also not in the more softer skills that you might get through research and development or investments in human capital. All of that is where you get benefits in terms of productivity. So yeah, the investment angle is certainly one where I see that coming through. I think it also makes firms and households more conservative. So instead of spending, they might be rather saving and that also creates a more austere environment for the economy. So those are a few ways in which uncertainty might be undermining economic growth.
Paul Barnhurst:
And I can definitely see that, especially the last one, right? We’re concerned so we save, we’re not spending the economy’s not growing it’s going to contract if everybody’s saving And so similar to businesses, they’re they’re concerned about something so they don’t invest. So speaking about uncertainty, what advice would you offer when to someone trying to reduce risk? I know we’ve talked a little bit about scenario management and planning, but what are some other things we can do to help reduce risk related to uncertainty or at least try to manage that because it’s never going to go away. We all know you can’t get rid of it. As I like to say, as I heard, I think it was George Box said, every model is, every model is wrong, some are useful, right? No forecasts perfect, but how do we balance that and manage the uncertainty in our forecasting?
Maura Feddersen:
So one of the things that you can do is to try to anticipate the types of decisions that might be required before you get to that point of having to make that decision. So thinking in scenarios is really powerful, but there’s also another tool. You mentioned earlier the post-mortem. So something’s gone wrong, the patient has died, why did that happen? And looking in the rear view mirror often it’s so easy to see. So instead of letting it get that far, we ask a question earlier on, which is for example, in X year, say in two years I will be proven wrong. Why might that be? So I’m essentially using prospective hindsight bias in my favor and research has shown that really unearthed a lot more drivers and blind spots than other types of questions about the future. So that’s really actually the power of negative thinking. So let’s say I asked that question and I realize I’ve missed something now that allows me to account for that directly rather than getting to that moment where I’ve been wrong and I need to think from scratch. So essentially it means that I can accelerate my decision making when we get to that moment where it’s really critical.
Paul Barnhurst:
I really like that way you mentioned the negative thinking and asking, okay, if this went wrong, what would be the main reasons? What would cause it? Because it allows you to one think of things you wouldn’t have thought about otherwise. Especially if you have a group that collective of well could be this, it could be that, right? You get a lot of different opinions. And two, there may be things you can put into your plan in case that does happen to help prevent it, it may change your forecast but it may also allow you to change direction. If turns out that one of those things you came up with all of a sudden looks like, oh that’s going to happen now.
Maura Feddersen:
Exactly. You might not have all the data yet to know what’s the scenario you are in, but at least you can think about what do I expect to see say one year down the line that would tell me what’s the scenario I’m in? So that’s the type of thinking that at least you might not be ready for the decision now, but at least you are getting yourself ready.
Paul Barnhurst:
Yeah, I really like that. I mean it’s something I wish I had used more in my career and I can definitely see where that would be really beneficial. So I appreciate you sharing that and that’s definitely something I hope all our audience starts using more in their forecasting because I can see where there’s a lot of benefit to that. Just like we talked about postmortem and scenario and other things as well. So can you maybe talk a little bit about forecasting at Swish Re? I know you’ve talked about, cause there’s kind of some best practice methodologies to use there that have been put in place to improve the forecasting experience. So maybe talk about that experience and how you’ve improved the ability for underwriters to be more accurate. Cause I know if you get it wrong, the policy, you could lose money, you can make money, it’s critical that you try to be as accurate as possible given the fact that there’s uncertainty in any of these things you’re underwriting.
Maura Feddersen:
So essentially this work happened, it started already in 2017, there was a team of underwriters that needed to forecast market trends. So do we expect premiums to grow up at what pace? What about severity frequency? So really critical assumptions and the data can give you maybe a partial answer, but the quantitative model alone was simply not enough. So they wanted to also leverage their expert judgment. And so here the methodology tested was really one that leverages individual thinking. So as an individual underwriter, I develop my own judgment first, then I bring in a group. So it could be a small group. They might have different views. So I get to benefit from viewpoint diversity and then I get to step away again as an individual underwriter and consider updating my judgment. So here we are finding a good balance between initially developing our own view , but then also learning from others but not falling prey to group think and some of the other group dynamics that might come into play.
And so that methodology was tested and the finding was that using this way of thinking could help improve forecasting accuracy but by at least 5%. And that was really convincing for the team and they decided we want to integrate that into our ways of working going forward. So initially with Excel, checklists and Qualtrics type surveys and now increasingly with a digital tool that allows us to bring all this thinking into one knowledge store. So you are guided through the process but you also have a space that you can return to go through your thought process again and learn from it because it is something that is a recurring exercise as well. So those are some of the ways also now that we’ve been spreading it beyond that initial team, that was essentially the testing environment
Paul Barnhurst:
Sounds like an exciting project and very rewarding to see how you’re able to improve accuracy by 5%. And it reminds me little different situation, but I remember in grad school we had this accounting assignment and I was convinced it could only be done in a certain way and we were talking about it as a group and everybody kind of looked at it before and pretty much got everybody on board with me that it just didn’t make sense. And then I went home and rethought about it on my own and I’m like, why was I that it has to be this way. And so I completely changed what I was doing and I think about half the class followed what I had said and when I changed it I was right. So I remember everybody was mad at me in the class and I had kind of dominated kind of been group thinking I was, oh yeah, no, that’s how it has to be. And then once I got back on my own and really took some time to think on my own, I’m like, that can’t be right. It makes no sense, it has to be this way. And completely changed my thinking and it ended up being correct and it just reminded me that importance of being able to step back so you don’t get in that group think mentality or that kind of collective bias.
Maura Feddersen:
And typically in an organization, in a team you’ll have someone who is maybe more senior, you’ll have someone who is vocal potentially maybe they also have an incentive to influence first and foremost. And so essentially if you at least have your individual starting point that is well-founded based on some of those ways of thinking that we talked about ranges, scenarios, you have something strong to go on and then if they have something else to add that’s helpful that you haven’t considered, you can incorporate that but it’s not going to dominate your thinking hopefully as much you can still structure the group exchange in a way to say, look, let’s be careful about some of these group dynamics. Make sure that we don’t, for example, share our estimates, but rather we focus on the underlying drivers, we make sure everyone gets to share their views and so on. So this is very common I’d say from what I’ve seen.
Paul Barnhurst:
Yeah, I think it is very common to have someone dominate the conversation often for various reasons. I mean I’ve been guilty of being the one in some kids situations that dominating and I’ve seen other times where others have dominated. So I would agree that’s all very common. Now kind of next question, what are you working on now? Is that project still ongoing, kind of improving the forecasting? Is there a next step you’re doing? What’s kind of your main project now that you’re working on in relation to all that?
Maura Feddersen:
It’s been a project that has really evolved and it’s been great to actually see it through for such a long time. So initially we were ramping up use cases and were keen to get teams on board. Then we looked at the toolkit, can we bring everyone into the same space to build that knowledge store? Also to start building a data asset where you are gathering all these forecasts, you are able to close the feedback loop because you’ll have the outcomes and then you are starting to create a better foundation for people to do their retrospectives because one of the findings also from the research is that if you give people feedback of how well calibrated they are, that already helps them adjust. So just looking in the mirror is useful here. And so the focus at the moment is really to grow adoption, to keep measuring where do we see improvements in forecasting accuracy and why? Where do we not see that? Can we make the methodology leaner? And so that’s certainly a focus and we are also looking at whether externally outside of Swiss, our clients are interested in this toolkit as well. So that’s also an exciting pursuit at the moment.
Paul Barnhurst:
That sounds really exciting. The external and see if there’s other clients and ways you continue to grow what you did. Sounds like been a very rewarding project as I hear you talk about it. So that’s great. Sounds like you have a lot of passion for it. Next question, you’re just speaking of best practice methodologies and forecasting. How do you balance the role of human judgment and experts versus AI machine learning algorithms, right? It’s kind of all the rage these days with chat GPT and now Bard and it seems like we’re every day hearing about new things with technology. So how do you balance those and how do you think they should work together, that human expert versus the technology?
Maura Feddersen:
Well certainly even artificial intelligence can be biased. I saw some interesting chat GPT stories where depending on essentially what you feed, Chat GPT defines also how it responds to you. And so I think it’s worthwhile even critically assessing whatever outputs we get from artificial intelligence and that’s where some of these tools that allow you to think critically come in really handy. So I am a big proponent of essentially bringing those two together, whatever the artificial intelligence suggests. But still keeping a role for humans and their judgment. But ideally you would give them the tools to think critically, is this output that I’m getting from this highly sophisticated model, does that actually make sense or is something not being considered here? What data did I feed it? Did this make sense? So that I think is a risk if you don’t do that, you might end up with some pretty, in hindsight, pretty obvious mistakes.
Paul Barnhurst:
Gr great point about challenging the assumptions. As you mentioned AI, how it learns depends on what you feed it and what you feed. It can be biased. It doesn’t mean that everything’s perfect that you feed the machine. And so I think there’s a lot of good lessons there. I appreciate that and I tend to agree with you, we’ve all, I’ve seen forecasts done by machines and there’s times you look at it and go, that can’t be right. So it’s likely why, what are we missing? What doesn’t make sense here? It’s just like when a human does a forecast, you have to review it and be like, sometimes I know I’ve got done with a forecast. And just from a sense check standpoint, it just logically doesn’t make sense. It’s like, okay, what did I miss? What assumption is there a mistake in my model? And you go back. I think that same process happens no matter what technology or what you’re using is you got to be able to challenge it and make sure you can be comfortable and explain why the number or the range of numbers make sense.
Maura Feddersen:
Exactly. You could try a devil’s advocate approach, ask all those difficult critical questions. You can put a whole team on it, a red team that tries to find the weakness in the model, in the forecast. So there’s some strategies that have been proven to really help identify what the gaps are.
Paul Barnhurst:
I like how you mentioned kind of red team devil’s advocate. I can imagine those really help because you get ideas that you just don’t think of all of a sudden you’re like, oh wait, that’s a good point. I hadn’t thought of that. I know I’ve been in meetings with management, well did you think about this? No. Well you do realize that’ll have a big impact. Oh, that’s a good point. Let me go back and add that to the model or adjust accordingly. So I appreciate that. A lot of great advice there and I mean it’s an exciting time to see technology and see it continue to grow, but totally agree with you there. There’s always the need for that human expert and that balance. It’s not one or the other. It ideally should be both together. So you had shared a quote on Twitter that I really liked. I saw it on your feed, I just want to kind of ask you about it. You said we can only approach an objective truth through debate. We can make up for our own reasoning errors through the collective if we engage critically with others and learn from others’ viewpoints. Can you maybe talk about that quote, what you would hope others learn from that?
Maura Feddersen:
I think it’s clear that we have a certain life experience, certain things that we’ve learned about through study through work and that is not all encompassing. So there’s bound to be other things that you can learn from others. And ideally you have a space where you can really critically explore what those viewpoints are, what the underlying reasoning is. Because in that way you can unearth really where the differences and where perspectives are coming from. So sometimes you can use even those tools around what was your lower case, what were you thinking about there? Why was your range so wide or narrow? Did anything come up for you when you were thinking about why you might be wrong? So you can really go into the details of your thought process. I found it helpful when talking to teams to encourage them to really put on the hat of being scientists.
So trying to objectively pursue essentially be truth seeking and try to put aside for a moment other considerations, business considerations. Let’s first find out what could be the truth and then we essentially react to that rather than combining the two in one sometimes. The other thing I found was helpful for teams is to use a fictional character called Rio. This is something that I know the University of Sheffield in the UK is using. Rio stands for a rational impartial observer. So this could be a creature that listening to the exchange and maybe there’s an intense debate happening and then instead of the group trying to come to a consensus at that moment you’d rather imagine, okay, this person, this creature has been listening to us, what would they say? As a rational, impartial observer. And sometimes that’s a very powerful way of having that critical debate. But then at the same time at the end, finding some sort of reasonable combination of those views and being able to take it forward from there.
Paul Barnhurst:
That’s so fascinating. I hadn’t heard of the that rational, impartial observer. And I can see where that’s a really good idea. Just have one more question then we’re going to kind of move into our standard questions we ask everybody as we wrap up. Is there any kind of book that you would recommend or a reading you like that really talks about seeking truth through debate? And this idea we just kind of talked about, is there any books that you find that you like on the subject?
Maura Feddersen:
So many obviously at top recommendation I’d say is Super Forecasting by Phillip Tetlock. So that really captures the research into trying to measure what really helps forecasting accuracy and being quite scientific about it at today. They are also other great authors. I would say also, I’m a fan of Annie Duke. She is a former poker player, turned decision scientist. And she focuses also a lot on closing that feedback loop between what’s your forecast, how accurate were you, and what’s there to learn from that. So those are just two rec recommendations.
Paul Barnhurst:
Yeah, she was on a podcast that Adam Grant does recently. Yes, and I remember listening to a great episode. She was very fascinating. So I remember thinking at the time, I need to pick up one of her books. I haven’t done it yet, but I remember listening to her on a pod podcast, Annie Duke. So thank you. We’ll put those in the show notes. So here we’ve moved to the portion of the podcast where we have some standard questions we like to ask everybody. And the first one we ask is, describe a time that you’ve had a failure at work, where you experienced a failure and what you learned from that failure. We’re big believers on the show that failures are just learning experiences. They’re a way for us to grow and learn. So maybe if you could share an experience where you had a failure and what you learned from that.
Maura Feddersen:
Yes, I had an experience where I received some data that I thought had been adjusted for inflation. So essentially became real price data, but that was not the case. So I started modeling and the numbers were, it seems all lining up, but then I realized something was off. And it turns out actually that data had received an adjustment that added inflation again. So we had essentially double inflation and I kicked myself afterwards. I thought I could have just done a spot check that this had worked, the adjustment. I could have definitely applied some critical thinking. So because of the time lost, it’s very much a stuck in my memory that at least I would learn from this experience. So yeah, I can share that.
Paul Barnhurst:
Yeah, no, I can relate. I still remember the time where when I was working, it was this rewards program and we had a very special way they wanted us to calculate everything that was different than what we’d done before. So I had to rewrite all the logic and in there I turned something backwards. And so it was giving me the worst performers, not the best performers. And I sent that out and we paid all of them as if they were the best. And so then I figured out after we had already paid everybody and had to go unfortunately to manage, I screwed this all up. And so we as a company had to pay for it. Cause we couldn’t go back to the vendor and say, oh, we paid all the wrong people. Can you double your money? And it was a really good learning experience of, okay, double double, triple check, make sure your logic is right. Go through and validate one of those all the way through and go, okay, is that really what should have happened? And yeah, it was pretty embarrassing moment for me when I was like, oh, we just spent $50,000 paying all the wrong people.
Maura Feddersen:
Oh dear. Yes. Even getting a second person to have a look. I think sometimes it’s comforting.
Paul Barnhurst:
It is. Sometimes it’s really good idea, Kate, can you just take a look at this and see if it makes sense to you? Because that often we get too close to the data. I know I do. Sometimes you just, you’re almost like you’re married to it, you’re working on it so hard and sometimes you just don’t see things that somebody with a fresh perspective can immediately look at and go, well have you thought about this? It’s like, oh, that’s a good point. So I agree. Really good advice there. So next question here. This is one we like to ask everybody. One of my favorite questions, questions. What is something unique about you that you can share with our audience?
Maura Feddersen:
I dunno how unique this is, but I certainly try to bring, they say don’t bring work home, but I actually like to apply some of these ways of thinking also in my personal life. So you’ll typically find me even asking outside of work what could go wrong? I assume something will go wrong, what could go wrong? And I find it actually not always appropriate, but actually quite helpful for avoiding various mishaps. So that’s one. The other thing is there’s something called the crowd within. So you might not always have that second person handy that you can pull in, but you can certainly sleep on it at night. Ask yourself again, ideally with as much a clear mind as possible. And I’ve tried to do that as well. So I use myself as a little bit of a Guinea pig I’d say.
Paul Barnhurst:
Well that’s good. I’m sure you’ve learned a lot from doing that and probably helped you with some decisions you were trying to make where if you hadn’t done that, you may have made a decision that would’ve been suboptimal, so to speak. So that makes a lot of sense. I like that. Next question here, do you have a favorite Excel formula feature or function? I imagine you use Excel a fair amount, and so is there a favorite thing you have about Excel that you like?
Maura Feddersen:
I think what’s actually maybe a slightly underutilized tool is to simply graph your data to begin with just to see what it’s actually looking like. Again, that spot check that we were talking about earlier you can do is this the data I’m expecting to see? And specifically I quite like a box and whisper chart. I know there’s that graphing functionality now in Excel, so you get a little bit of a sense also of the distribution, what’s the middle value, what’s sort of the range. That’s really powerful. So I recommend always doing that.
Paul Barnhurst:
Yeah, I had a CFO and it’s really taught me the importance of it all the time we’d run, be running through some assumptions. Can you graph this? Can you graph that? And you wanted to see it visually and be able to, doesn’t make sense. Does it hold together or is there some outlier that I should be concerned about? And that was a really good lesson for me of just the value of graphing something because there’s times we graphed it and it’s like, yeah, that doesn’t make sense, does it? And he’d be like, no, it doesn’t. You should have caught that. Yeah, you’re right, I should have. And
Maura Feddersen:
It’s pretty quick, right?
Paul Barnhurst:
Yeah, exactly. It usually takes a couple seconds to throw it in a graph, especially if you’re dealing with ti any kind of time dated, it’s extremely easy. It doesn’t take much to look at a quick trend and say something’s amiss here and it’s much easier for eyes to catch. I teach in visualization courses now. That’s one of the things I do. I have a data visualization course and it’s something we talk about is you really want to make it clear what people are looking at and things will jump out if you design it. And just, I think when you’re doing exploratory, the same is true if you do a quick graph that graphs the data in the proper way. If something’s way out of line, it’s going to stand out. If there’s something small, you may not notice it depending on the size. But I agree. That’s a great, I really like that one. We haven’t had that answer before, so that’s good. Good. Got a first for us. So we have just two questions left here. First is if someone was starting a career today focused on forecasting, whether that be FP&A forecasting underwriter, whatever it might be, what’s the advice you would offer to them?
Maura Feddersen:
I would recommend covering both the domains of quantitative as well as qualitative in a balanced, holistic way as much as possible. I do think people tend to have a leaning towards one or the other. Maybe they feel that they’re able to do one more easily than the other. Sure. I would say try really to emphasize both. I think data science is still a very hot area. I think it will remain so and it’s just worthwhile also applying some of the best practices for more qualitative judgment as well, and making sure those two areas are really strong.
Paul Barnhurst:
I like that, finding that balance. And I agree with you. I know I tend to lead a little more toward qualitative than the quantitative, but you got to have both. So that makes a lot of sense to me. And then the final question, if somebody wants to learn more about you or connect with you, what’s the best way to do that?
Maura Feddersen:
You can find me on the usual social media channels like LinkedIn, Twitter, or you can pop me an email. So happy to share my email address with you, Paul. So yeah, those are two good channels.
Paul Barnhurst:
Well, thank you. I appreciate that. I appreciate you taking the time to meet with us today. I think it has become dark as we’ve been talking, so I’m sure you want to get home. But thank you very much for carving out some time for Mario. I really appreciate it and I thought you were fascinating to learn from. I love getting that different perspective of behavioral economics because there’s a lot we can bring to that as FP&A professionals. So thanks again for your time.
Maura Feddersen:
Thank you, Paul. It’s been a great pleasure. Thank you.
Paul Barnhurst:
Appreciate it. Thank you.