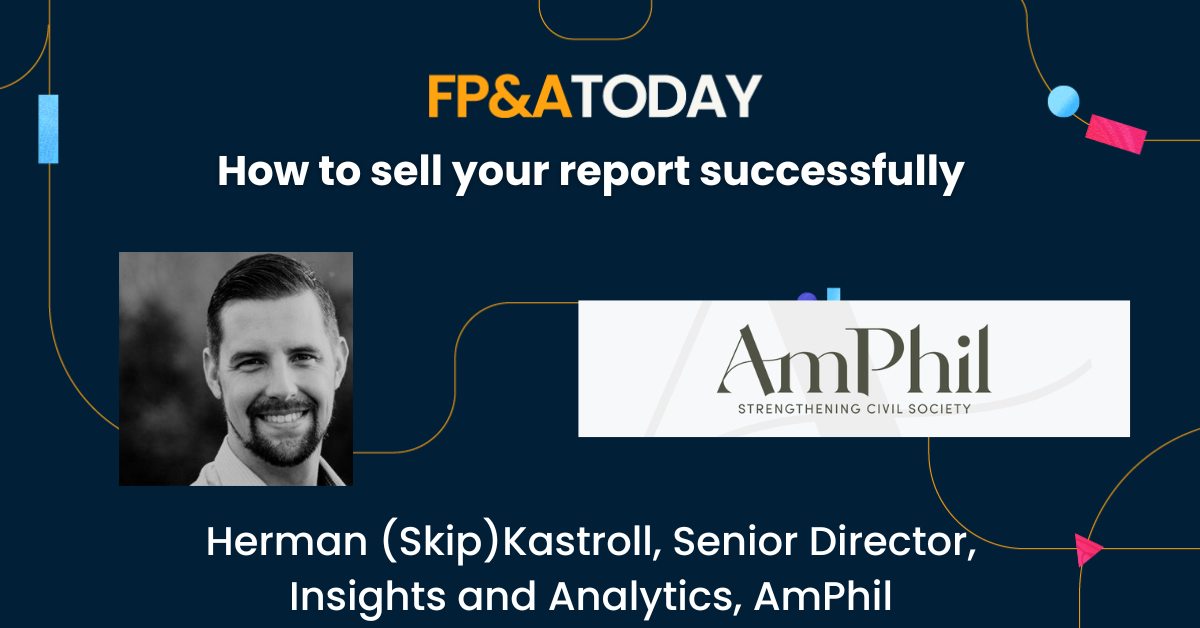
Skip Katroll is Senior Director, Insights and Analytics at AmPhil. AmPhil helps big-name nonprofits raise more money and advance their missions. This episode is packed with practical and honest insights and takeaways you can apply to your day job. Skip gives the inside story on creating and selling a report at Amphil, “Unlocking the Secrets of Lost Sales Opportunities”, and the approach that he followed. Skip also reveals how a background leading finance at top universities alongside math, economics (and a love of sci-fi) informs his FP&A.
Prior to joining AmPhil, Skip was an associate vice provost at Liberty University responsible for the institution’s strategic planning and regulatory compliance.
In this episode:
- My multidisciplinary journey (aka “confused youth”) – studying math, economics, educational leadership and religion (and how it applies to my data insights)
- FP&A business partnering at a University Finance team – navigating functions from the Dean of Arts to the Dean of Engineering
- 3 proven strategies for financial data and analysis getting management to informed decisions
- Countering “so what, and “why am I am here” approaches from business partners
- How to navigate ad hoc requests
When you don’t have enough access to data - The power of benchmarking
- Leading vs lagging indicators
- Unlocking the Secrets of Lost Sales Opportunities – a big impact report and the challenges, impact and approach
- My favorite Excel function
Connect and and ask questions to Skip over on LinkedIn: https://www.linkedin.com/in/skipkastroll/
Further Reading:
Skip Kastroll: Bridging the Gap: Translating Finance Insights for Effective Decision-Making
Full transcritp below
Glenn Hopper:
Welcome to FP&A Today, I’m your host, Glenn Hopper. Today I’m thrilled to welcome Skip Kastroll to the show. Skip is the senior director of Insights and Analytics at Amphil, where he supports the consulting groups with project management engagement reporting, and long-term planning. He’s an expert at finding and untangling disparate processes to drive optimal efficiency. Prior to Amphil, skip served as an associate vice provost at a large university, overseeing strategic planning, regulatory compliance, and the evaluation of over 500 academic programs and business units. His background spans, systems implementations, project management, and training. Skip’s educational journey is quite unique. He holds a bachelor’s in mathematics and Economics from the University of North Florida, a master’s in religious education and a doctorate in education from Liberty University. Talk about a multidisciplinary perspective. In a recent article for Data Rails, skip shared his insights on how finance professionals can communicate more effectively with leadership. We’ll be diving into those key tenets today, as well as exploring skip’s thoughts on leveraging analytics to drive better business decisions, building trust in data and upskilling for the future of FP&A. When he’s not untangling business processes, you can find Skip riding his bike on the road, the trails, or wherever has the most downhill. Skip, welcome to FPNA today.
Skip Kastroll:
Thanks so much for having me, Glen. I’m excited to chat.
Glenn Hopper:
Yeah, I, it’s gonna be hard not to just talk about cycling this whole podcast, I think
Skip Kastroll:
<Laugh>. Yeah, that’ll be episode two where we talk about cycling and FP&A
Glenn Hopper:
Right. Perfect. <laugh> and how we justify buying that next bike with complicated formulas and FP&A<laugh> project. Exactly.
Skip Kastroll:
Exactly.
Glenn Hopper:
<Laugh> this, the formula is simple, though. The number of bikes you need is the current number of bikes you have plus one. Yeah,
Skip Kastroll:
The n plus one, I’m, I’m having a hard time getting my wife to understand the the science behind that, but I keep repeating it, hoping it’ll stick.
Glenn Hopper:
So I really appreciate you coming on and, and sharing your insights and your insights. You know, I, I, I talked about it in the, in the intro, but you have such an interesting and diverse background spanning mathematics, economics, religious education, and education leadership. So I’m wondering, you know, everybody kind of brings their own insights and, and thoughts in, into the job, but talk about how your multidisciplinary journey, how has it shaped your approach to data analytics and communicating insights?
Skip Kastroll:
Sure. my multidisciplinary journey, which you mentioned, which also sounds much better than confused youth has, has pretty been pretty pivotable in shaping my approach to data analytics and how you communicate those insights. So each field that I’ve studied has contributed pretty unique perspective that I apply in my work on a daily basis. So my foundation in math has provided me with this idea and this concept of analytical rigor and logical thinking, which is pretty essential when you’re working with increasingly more complex data sets, helps me approach problems systematically and in helps with the accuracy. Economics has taught me to consider the broader context of data, understanding not just the numbers themselves, but their implications for decision making, resource allocation. And this has been particularly helpful when you translate that data into actionable things. My studies in religious education and education leadership have been pretty valuable in developing a more holistic view of how data is organized and how it fits within an entire organization.
So these fields have kind of helped emphasize the importance of understanding diverse perspectives and the human element in decision making. So, I guess an example in my leadership role in institutional effectiveness, I often had to synthesize complex data analyses within the practical needs of various and different stakeholders. So that experience taught me the importance of aligning insights with organizational goals and strategies. Also my the, the journey of my educational and work journey kind of has instilled a, a good appreciation for the interconnectedness of different fields. So when I worked in a university, I would often work with the dean of the School of engineering and then the dean of the arts department, who are two very different people. So I found that insights from one area or one discipline often have unexpected applications in another, so you can kind of find more innovative and comprehensive solutions. And I use this at how me to approach many different client and internal challenges from multiple angles, thinking of rigorous data analysis, kind of with a nuanced understanding of organizational dynamics. So I, again, just to go back to being, I like multidisciplinary jury better than confused youth. But I think the confusion has kind of wrapped itself around kind of a more holistic and bigger picture. When I think about data and analytics,
Glenn Hopper:
I love that. And that’s a, that’s a key point too, because we can get so lost in our models and in, and the data. And when you forget the, the human element of it, you know, when it’s just numbers, it’s easy to overlook things. And when you, you know, and I think, not to go off on this tangent too far, but I think business partnering, which is the buzzword that’s been going around FP&A for the past several years, is really a, a big part of that too, because you’re, you’re interacting with the other departments and knowing that, you know, these numbers mean something more than just whatever appears in the, in the general ledger and whatever appears on, on a budget. So having that approach, and I also in, in so many ways, that math background is so key because there’s a, an old meme about, you know, like your understanding of calculus or, or statistics, you know, when you’re in school, and then what happens when you get in Excel <laugh> and you just simplify everything down.
So having that base in economics, and actually the base in economics is huge too, because when you’re modeling and, and forecasting, it’s easy to just get hung up on the internal data. But there are all these exogenous factors and things that happen outside of what you’re doing that you have to factor into your forecasting. So having that economics view with the, the good math and knowing what makes a good statistical model and knowing what features to picks, or I guess in FP&A terms, it’d be more what your assumptions are and what the drivers are and all that. But it’s just having that broad scope really brings a lot to the field. And whether someone has a, a classical education in the field or not, they’re all things that need to be considered in your, in FP&A.
Skip Kastroll:
Absolutely. I’ve worked with really smart, intelligent, and well communicating folks that have had their discipline all the way through math and finance. And I’ve also met folks like myself that have kind of bounced all around. So I think you can build that well-roundedness in a bunch of ways.
Glenn Hopper:
Yeah. Yeah. So you and I first got connected because you wrote an article for Data Rails and in this article, and we’ll share a link to the article in the show notes too. But pretty interesting. You discussed three main tenets for finance professionals communicating with leadership. So I think this goes back to your multidisciplinary approach because, and, and honestly, in FP&A now I’m seeing more and more people talking about the art of storytelling and how important it is. You may understand exactly what’s going on. You spent 25 hours building some super complicated model, and you, you love the results that you’re getting from it, and you, you trust it and all that, but if you can’t explain that to people, all that time is lost. So tell me a little bit about the article. Give, give our listeners a quick overview of those key points that you talked about in it.
Skip Kastroll:
Sure. Happy to basis on three tenets or three strategies, and I’ll just kind of give the quick overview of each one. So first strategies focus on keeping it simple. This involves kind of identifying the core message and explaining it in a pretty clear and concise manner. Kind of focusing on what management or your c-suite or your executive team, kind of what they care about most, which is most often whatever the trending KPIs are, whether they’re yearly KPIs or they’re just standard KPIs. And thinking about how this has the greatest potential impact. This doesn’t mean oversimplifying complex issues, so you can’t oversimplify like a, a multivariate analysis. It, it is what it’s but rather, rather it’s presenting the information that’s easily digestible for non-finance folks. So strategy number one is keep it simple. Strategy number two is make it practical.
I’ve found in the many years that I’ve been working that when people are pressed with time, they will al almost always fill their open time with what is practical over what is ideal. Not all the time, but that’s usually the, this balance that they strike. So if you can make your recommendations practical, they will often lean towards your recommendations. So you also have to factor in other things like, you know, budgetary limitations, resource constraints, different organizational priorities. So you have to balance your insights with the larger picture of what you’re working with so that it can be practical. When I worked in education, people often wanted to just revamp the entire system. And while that sounds great and it might be needed, it’s just not practical. You can’t go to a provost or a CEO of your organization and say, next quarter we need to redo everything and expect them to just like shrug their shoulders and say, sure, let’s do it.
Let’s do it. Now they might, but it’s not often gonna be the case. So second strategy is make it practical. Third strategy is to learn to differentiate, but what about what is interesting and what is actionable? So often finance folks will find ourselves, and you mentioned this earlier, some like fascinating analysis that we’ve done but it’s really helpful to focus on, are those insights that you’ve found or are the insights that people are asking you to look into, are they interesting or are they actionable? Ideally they would be both. But I often try to remind folks that interesting work that isn’t actionable is oftentimes just more work. It can turn to be actionable, but if the intent is not that it would be actionable, then it is a little tough. So, so I argue that if you apply those three strategies, it can really help you communicate what you found up to your c-suite, your executive team, or even your colleagues that are working with you to hark back to what you said, kind of put yourself as a strategic partner in the business and success of the business as opposed to just the number crunch or just the data guy, or just something else.
Again, it’s not, it’s not just simplifying complex information, but understanding your audience and how you can align that with organizational goals.
Glenn Hopper:
Yeah, and, and I really I wanna dive a little more into the, the storytelling part because it’s, you know, like you said, you, you could have, you could have some fascinating insights through some complex math that, that you were, you’re doing a multivariate analysis or, you know, some kind of model that you had to put together, you know, all this different information. You’re getting information from the, the sales team, from the operations team and all that, and you’re aggregating it and you’ve done your statistical analysis and you’ve figured out all this stuff that it’s your domain expertise that puts it all together. But you want, you know, you want to have whoever you’re presenting your story to, you wanna have them, them understand that you are an expert at this and, and why you did it, but you also don’t wanna bog down in, you know, why you chose which specific <laugh> statistical model to, to come up with your results, or, you know, why you chose whichever data point you need to, if the questions come up, obviously you need to be able to answer ’em, but it’s hard. I, I think to find that balance between giving the message, giving the recommendations, showing that it’s not an opinion, that it’s based, you know, it’s, it’s data-driven. Like how do you, I I think that we, a lot of us are challenged with that. Like, how do you get that story across better when you’re talking to this c-suite? What, what tips would you have for analysts who are trying to improve? You know, they, they may be the best analyst in the world, but they really need to improve those data storytelling skills.
Skip Kastroll:
Sure. I’ve got three thoughts, and then a random sidebar that came came to me the other night. So a couple of initial thoughts. You, you need, you really need to know your audience. Who are you presenting it to? What do they care about? That’s not always easy. So you can ask them those questions prior to the meeting or if you know them, but know your audience so you know who you’re talking to. It’d be akin to like writing a kid’s book and expecting like high schoolers to read it. It’s just you, you need to know your audience, who you’re, who you’re writing to. Second start with the why. Almost all meetings that I’ve had with c-suites or executives, their first question in their mind, whether or not they actually say it out loud is so what? Like, why am I here?
And if you can address that, why, or that’s so what, early on in your presentation, you can usually draw them into what you’re, what you’re doing. Thirdly, use visuals effectively. I’ve seen some superb graphs and charts, and I’ve seen some that actually make me dizzy. So learning how to make well-designed charts and graphs so that people have a memorable visual element that they can take away with the meeting. There’s a ton of great books on there that you can use out in the, out in the, you know, world of things to help you find a great way to do it if you don’t know. But really use visuals. So there are, I guess, three tips. And as you and I like, touched on this conversation and a a couple of weeks ago when we were chatting, I just kind of kept thinking that, while those are great tips, I mean, I, I said them, so I think they’re great.
I think there’s gotta be, there’s gotta be a little bit more to it. So I don’t know if you know this about me or if we chat about it, but I’m an avid sci-fi and fantasy reader. I love the books. I read American Sci-Fi, Eastern Asian Sci-Fi. I read tons of Sci-fi and Fantasy. And the other night I, like, I cracked open my nightly read, I cracked open on my Kindle, like I flipped it and I turned it on <laugh>. And I got to thinking like, what, what is connecting me to this story? Like, why do I keep opening, why do I read this for 30 minutes to an hour every night? And I realized that there’s a lot of emotional elements that make a good story memorable. And I was thinking like, why do we shy away from this when we’re talking about data storytelling?
I mean, you think of some of the timeless stories in literature like The Hobbit 1984 To Kill a Mockingbird, or Who, whatever your favorite story is. Like, they resonate with us, not just because of their plots, though, they’re good, but because kind of the emotional journey that they take us on. I mean, I’m trying to imagine the Hobbit, if it was written simply as like bilbo’s itinerary, like, I went here, I did these things and I met with these people, like that would be just disturbingly boring. But you know, when we think of data storytelling, we often shy away from emotion thinking it’s gonna like somehow undermine our objectivity. But I think if you can incorporate like an appropriate emotional element in there that’ll make our stories a bit more compelling and also more memorable. And I guess the key is to find the human element.
Behind every number is a customer, an employee, some business outcome that you can approach that affects real people. So you can highlight these connections and kind of create emotional resonance that makes these insights stick. That doesn’t mean becoming like an actor and overplaying and fabricating drama and overplaying into emotions. There’s certainly dangers on each side of the extremes. But I think if you can find the genuine human impact that the data is letting you guide the narrative, that can, again, make it memorable.
And I think for me, that’s, that’s what’s important about the communicating these insights, is you don’t want folks to leave the meeting, leave the presentation, and go five minutes later like, well, what did Glen say? What did Skip say? Like, I kind of forget, you want something that sticks them back and kind of brings them through to it. So just kind of a, maybe a side tangent that I thought is, is, is missing in a lot of data storytelling.
Glenn Hopper:
Yeah, it’s, it’s funny as you were saying that. So a couple of of thoughts came up. So first off, when you were talking about I was looking for my Tufty book, but I’ve got <laugh> for visualization. But then I was also thinking about a lot of these books back here are, these are not all business books. I’ve got the collected works of Philip k Dick down there, Kurt Vonegut’s, right there, Richard Brogan Cormack McCarthy <laugh>, you know, but I, but it’s funny, when talking to finance and data people, how many of us are we’re avid readers also, so you’d think like right brain, left, left brain, they would be separated. But the, the marriage of storytelling and the data, I, I think being able to communicate that they really do go hand in hand. So what’s amazing to me hosting this podcast is how many finance people turn into writers, how many finance and data people turn into writers?
And as you were going through and talking about, you know, if The Hobbit was, if it was just an itinerary, I was thinking of so, you know Joseph Campbell had the Hero’s Journey that, you know, goes across mythology, but there’s also a book, Christopher Vogler, I think was the author, but it’s called The Writer’s Journey. And this sounds like the weirdest financial advice I’ve ever given. But in that storytelling, you know, I, you’re not gonna <laugh> do the full hero’s journey in it, but it’s a, a great breakdown of, of why people latch onto stories. So that’s a, a really weird obscure read, but I figured you’re probably familiar with your diverse background with some of that, but it could be factored in into the storytelling.
Skip Kastroll:
Absolutely. Yeah. The book that I’ve been using recently is, is Dona Wong’s, this Guide to Information Graphics. It’s it’s really nice. It’s, it’s pretty short. And you can kind of just cover through it. It’s a nice reference guide. So whenever I make a chart, I’ll always flip through a couple of pages on those and to gimme some good helpful tips there. Right before I crack open another fantasy read.
Glenn Hopper:
Yeah, right, <laugh>, right, so, alright, so we also, we, we talked for a, a good long while before the, before the show, and one of the things that came up, and I don’t even remember in what context it did. Oh yeah, I do. So you would, when you were talking about these projects that we can take on, because when you’re surrounded by data, whether it’s financial data, operational data, whatever it is you could report, you could do, you could p hack your way through and, and find all sorts of spurious correlations and all that. You could, I mean, you can go through and, and do this research or you could have some executive think he wants you to do, he or she wants you to do some kind of research that doesn’t really, you know, it’s an ad hoc request and you’ve got all the, all your kind of monthly, you know, you’ve got your your scheduled work you have to do, you’ve got your project work you’re doing, and then these ad hoc requests come in and you said something that I thought was really interesting. But as I think about these ad hoc requests that come in from leadership and how much of our time they take, how do you recommend navigating those requests and and, you know, determining which ones are are worthwhile?
Skip Kastroll:
Sure, I get those all the time. I got one of those earlier this morning. And it was a, it was a pretty good request. So my approach to ad hoc request is generally one of open communication and what I’ll call strategic questioning, which to be fair, not a lot of people actually like to have happen to them. So but one of the first things I do is when I get a request is I usually two things. One, if I’m about to ask someone a bunch of questions, especially a super, a supervisor or superior I always kind of preface them, letting them know that I’m about to ask a bunch of questions, which sounds silly, but I’ve found that over the years, people when they get, you know, machine gun questions, they often feel like they’re being questioned and nobody really likes that or being interrogated.
So I usually start out by saying, Hey, I’m about to ask a bunch of questions. I’m not questioning the request itself, but really looking for clarification. And that can kind of disarm some of that initial defense. So but one of the key questions I always ask is some version of how will this report, how will this request tie into a key objective at our organization? So like, how, how is this report gonna tie into our revenue goals for this year? Or how is this gonna tie into any, some cost saving initiative that we’re doing? Or increased hiring or expanding the marketing firm, something along those lines. So that does two things. It helps me understand kind of the strategic context of the request, and it often prompts the requester to think a little bit more deeply, or like the intent of the report’s purpose.
So I found that if that question gives them pause, it’s often a sign that we’re dealing with probably more an interesting rather than a useful report. So so if you’re like, how is this gonna be used? And they’re like, you know, it’s I thought about it. And sometimes they’ll derail and they’ll take back the request and they’ll say, yeah, that’s not super important right now. We’ll deal with that later. Which is helpful. So if they’re kind of reluctant to engage with that question or they don’t like it and I’m often really busy as most of our folks are, you listen, aren’t all listeners. I’ll usually frame it in some kind of utilization context. So I’ll say something like, more than happy to work on this report. I think it’s a great idea given some of the other requests that we’re currently handling that likely that person has also asked you to do, how would you like me to prioritize this one?
So that kind of acknowledges their requests, but also like gently reminding them that you are a finite human being with a finite amount of time. And it’s not really to discourage their request or to like boast about how busy you are to them. It’s, it’s really that oftentimes leadership isn’t always aware of how much time goes into some of their requests, how many requests they’ve actually given you. Like, you’ll not find many leaders that say like, I know that this past week I asked you 15 questions. They don’t keep a list of those. So and we don’t really need them to. So it helps them see that, like, what, what is the context of it? And then if there’s a bunch of other things, you know, you’re able to say, Hey, you know, I’m happy to reprioritize this, you know, that’s gonna, like, I’ll, I’ll do this other requests next week.
And if they don’t like that, then they’ll help you with that prioritization. So, so a lot of questioning, a lot of clarification and go from there. So the idea is not to dismiss the request outright, but get clarity and, and insight to it. Sometimes they’re just gonna ask you to do it anyway, and you know, if you’re listening to your boss, you’ll probably do it. But this habit of asking those same types of questions they’ll start to predict those questions when they come to next meeting. So they’ll say, Hey, skip, I’d love for you to do this request. It’s tying into our revenue goals. And I know you’re working on this other project, if you could prioritize this one to the front. ’cause The CEO really wants its information for a stakeholder meeting next week. And then I’ve got context, he’s got context, and we’re kind of on the same page.
Glenn Hopper:
FP and a today is brought to you by Data Rails. The world’s number one fp and a solution data rails is the artificial intelligence powered financial planning and analysis platform built for Excel users. That’s right, you can stay in Excel, but instead of facing hell for every budget month end close or forecast, you can enjoy a paradise of data consolidation, advanced visualization reporting and AI capabilities, plus game changing insights, giving you instant answers and your story created in seconds. Find out why more than a thousand finance teams use data Rails to uncover their company’s real story. Don’t replace Excel, embrace Excel, learn more@datarails.com.
To me, financial analysts we’re like the OG business analysts. Like we, we were doing this stuff before sales and marketing, got into all their machine learning and clustering and all the stuff that they’re doing. But also we seem to operate in a more finite world of data where we just think about the GL maybe the pipeline a little, you know, depending on, on what our particular role is. I think we sometimes think, oh, well it sure, it would be nice if I had all the data that sales and marketing did about, you know, who came to the website and what this purchase behavior was and all, all that. And we, we really, there’s a tendency sometimes to limit ourselves to just the, the dollars and cents to the numbers. And so when I talk to a lot of fp and a people the feedback I’ll get as well.
Yeah, we just don’t have enough data to glean meaningful insights beyond, you know, we’re doing our basic variance analysis, we’re taking the data we have and building our budgets. But I, you know, I think that there’s always additional data out there, especially, you know, we’ve been talking about digital transformations for 30 years now, and everything certainly has become more digital. So outside of our ERP or, or certainly outside of our gl there is other data out there that maybe we’re not being, that we’re not thinking about using. But what, I mean, what would you say to someone if they said, you know, I just don’t have access to enough data. All I can trust is what’s in the gl or what’s in the, you know, the income statement, whatever. What would you say to them to encourage them to do a deeper dive into, into data?
Skip Kastroll:
Sure. I would and like really just encourage them to, at the start, is that they probably have more data than they realize. And once they see that, they’ll probably have more data than they know what to do with. And if they don’t have enough data, like if they’re just looking at just the GL and that’s all they’ve got, I’ve not found a lot of organizations that are shying away if you ask for more data. So you can go to the marketing department and you could say something like, I know you’ve done, you know, 10 campaigns over the last three months. Could you just send me the listing of participants, types of people and, you know, the date stamps and any other relevant pieces of information. And then I’ll do the rest of it, I’ll, I’ll see what I can do with it.
And then tying that in with business outcomes. Like, did revenue go up in one month after you did a certain campaign? Like you can usually make some of those tie ins yourself, but usually people, if you ask them for data, they’ll, they’ll probably just give it to you. Now that’s not the same in every organization. If you’re working on something highly proprietary, they’re probably just not gonna dump everything to you. But usually you can just ask for more data and you’ll eventually find that you’ll, you’ll soon be swimming in data. You’ll just be like, you’ll wake up in the morning and just like, move spreadsheets around on your desktop. And so then you’re gonna have to know like what are you looking for? Where are you gonna find it? How are you connecting different BA data points? And like, what insights are you gleaning from those?
So another method I found that is really helpful is if you really don’t have the data that you think you need, if you go to a supervisor that you’ve presented the, like a GL report and say, and ask them like, Hey, when you look at this report, what do you wish you knew more about? And allow them to answer that question for you. And in my experience, they’ll most always give you more questions than you have time to answer. But they’ll help you kind of dive in and clue you into what data you need to analyze. So, you know, you may see someone, they may just point at a number on your report and be like, this, this one really confuses me. Why, why is this number different? Or, I thought this would be higher or lower. What’s the case here?
And they’ll often do that, like squinty eyed, confused dog look where they turn their head sideways when they point to it. And that’s just gonna clue you in on what’s more data that you can get, and that’ll provide value because you can provide an answer to their question. So maybe like monthly expenses went down because it was the end of the quarter and we know that next quarter they’re gonna go back up and you can provide that answer and, and frame it that way to them. So I think those are some great ideas. If you have some data, then you can build into it. So if you do like a quarterly business report, then you may think that’s all I have is a quarterly business report. Well, well after a year you have four of them. So you’ve got four data points that you can trend over time.
And each one of those pieces of each one of those numbers in your quarterly or business report is now trending over time. So you can build your own Excel reports based on, you know, PowerPoint presentations that you get. So you can build some things like that and kind of like foster your own curiosity and then, you know, look for correlations, segment the data trend it over time go into Google and benchmark that against some industry standards. Like what are other SaaS companies doing and how, how do they relate to this KPI for us, you’re, you’re gonna find loads of stuff out there on the big wide web about some information that you can benchmark against, and then you can certainly leverage tons of technology. There’s loads of tech tools out there that you can use to analyze the data that you have and kind of may uncover the insight. So engage with leadership and ask the right questions. I think are two main things that I would encourage folks to do if they’re kind of feeling stymied by the, the lack of data that they’ve got. Yeah,
Glenn Hopper:
I I love the recommendation on benchmarking too, because I think benchmarking, it’s, it’s almost the, the gateway drug that’ll, that’ll get you in be to using, you know, external data. Because if you, you know, if you’re, maybe you’re a a, a privately held company, but if you’re in the same industry as a public company, and, you know, so benchmarks can be hard to get, you know, unless you pay some firm to go get, get them for you. But if you are you know, a private company and they’re, you’re in the same industry as a public company, you can go pull down their, their annual financials, their 10 k and and all that and, and start comparing yourself to them. Now, obviously you probably don’t have the scale that they do, but there’s some, some probably some good numbers that come from that. And then taking like macroeconomic data and putting it in and saying, okay, well, you know, we, we’ve been around for 15 years, let’s look at interest rate over the time and how that impacted our, you know, our, our sales because we do, you know, a lot of loans or whatever, you know, whatever the data is that’s out there.
And then finding correlations between, maybe it’s internal data, maybe it’s external data, but that’s, that’s always interesting, especially, you know, the, the secret you wanna do if you’re in time series analysis is if you could find a consistent leading indicator. I mean, that’s, you, you’ve, you’ve found the magic wand right there, <laugh>.
Skip Kastroll:
Yeah, it’s, it’s so much fun to find the leading ones. Lagging ones are fun, but not, not as fun. It’s great to find the leading ones so you can course correct as you go along. And then you can norm yourself against, again, like you said, like competitors. I remember I was doing end of course, survey analysis at a university, and all of the professors were getting pretty high scores. Most of the students were saying things like, you’re rating them high on a Likert scale, like four or five. And everyone’s like, you know, almost all my students gimme four or five. Well, we eventually normed the instructors against the entire university’s data set, and we found that if you weren’t actually getting almost all fives, you were well below average. And so all these professors for, for quite amount of time, were thinking like, 80% of my students are giving me a four or five, like, I’m doing really good. What we found that that was, that put you in like the 10th percentile and compared to what other instructors are doing. So that was kind of an eye-opener for a lot of folks when they were able to norm themselves against their peers, so against people in the same industry and see how they compared against it. So that’s more with internal data, but the idea of norming, your benchmarking yourself is it can be pretty powerful and enlightening in good and bad ways.
Glenn Hopper:
I bet that could be used in HR also, where I think different companies have different cultures around the annual evals and you know, so it could be at this company, the culture is everybody gets pretty close to the three, you know, the, the middle of the range. But in this one, everybody, if you don’t, it’s the same. If you don’t get a five, then you’re <laugh> Yeah. Then you’re doing a terrible job. So you know, that would be, that would be another probably a little bit a field of what we we do in fp and a, but still it’s a lot of data to look at <laugh>. Yeah.
Skip Kastroll:
Another question to answer. So,
Glenn Hopper:
You know, and I think about this, the more we pull data in, and the more we start using more DA data, and the more complex our models get, like if you’re doing, you know, linear regression <laugh> to for your forecast, which I don’t think anybody’s really just doing straight that anymore, but a very simple approach, very small amount of data is very easy to explain. But the more you start pulling in data from other places in the company, and the more you start pulling in external data and the more complicated you make your models, maybe they’re getting more accurate, maybe they’re getting precise, and you can build up this trust over time. But if you’re talking about changing the way that you’re forecasting by adding more data, I can see there being resistance to it or hesitancy, like, how are you even gonna trust this data?
So, you know, as a data guy, you’re, you’re, I’m sure you’re always looking for the best data from, you know, as, as much of it as you can get. But when you incorporate all this, I’m assuming you’re not just doing, you know, you’re probably doing some machine learning models and some more opaque, you know, harder to explain models unless you’re talking to another, a data scientist. So how do you, when you have this complex model and you’ve really got the results, and I know we talked about the, the storytelling part of it, but there’s also another component of that is like, look, I get you weird stats guy <laugh>, but you know, you trust the numbers, but I don’t know that I do. So do, are there strategies or approaches you take to help the stakeholders kind of build that trust in, in data, especially for the skeptical ones, maybe more old school and you’re like, I don’t know this, and you know, here’s the CAPM formula, this is what we do.
Skip Kastroll:
Sure. So the approach that I take often, it kind of depends on where on this like journey of data trust. The person I’m talking to is I’ve found that there’s a lot of folks that are skeptical and then there are other folks that have a blind trust in whatever data is presented to them. And there’s, you know, again, danger in either one of the extremes. Like you mentioned, more often it’s the skepticism that we need to address. So I think the key is to start small and build incrementally. Like I think of like the analogy of like Legos, like when you’re building a Lego model, like, you know, small piece after small piece and then it turns into something that’s great. The analogy there is also is it’s really easy to break a Lego set. If you’ve ever like washed a 10-year-old crumble some Legos in his hands ’cause he broke, ’cause he got one piece on there you kind of know what I’m talking about.
So start small, build incrementally so you can have this foundation of trust and you should always have like a ready answer. Someone is gonna ask the question like, how do you know that this data’s accurate? How do you know that it’s complete? And, and you should have a pretty clear, concise answer ready, because what they’re gonna think is that if, if the data guy can’t answer that question, like, who, who can? So why should others trust it if you can’t even explain it? So a couple of best practices that I’ve used over the years is transparency. Be really open about your data sources, your collection methods, and any limitations or assumptions in your analysis. So if you’re pulling information from sales opportunities and several of the fields are human key punch information you can, you can say like, you know, this is hand keyed information and something might be misspelled and that may impact the analyses.
Maybe you did took care of that in the data cleanup process, but there are gonna be some limitations and assumptions in your analysis and be open about those. Be consistent. So consistent definitions, consistent metrics across the reports that you use. Your leadership is often inundated with terms information. And the more that you can make your stuff as much as the same across those communications, it’s just gonna be really helpful. And if you change some calculation method, especially if it’s one that’s been used for a long time, be pretty clear about why and how you did that. Do a lot of validation, a lot of cross-checking of your sources when you can. Is it aligning with other operational metrics? And if it does, that tends to build confidence with it at an element of storytelling. We talked about this and I think it’s a great point.
And connect it to like real world outcomes. You’re gonna be wrong at some point. And if you’re wrong, just admit that error and correct it. You know, not all of your analyses are gonna be perfect, accurate, and someone is gonna find something that just went right past you. You likely work with smart people and they’re gonna find errors that you didn’t. And then again, be transparent about that. Explain what happened, how you’re gonna prevent it in the future. Engage stakeholders in it. So instead of just presenting the data and walking away, like, don’t do like a mic drop, report drop and just walk out of the room. Like you just bring them into it. Like ask them questions. If you, if you haven’t found out yet, that’s my favorite method, ask people questions, you’ll, you’ll learn a lot. And then I think if you can bring in some data education to the organization you’re working with that will help stakeholders understand the data.
I think we as data folks often assume that people know a lot more about data than they do. And that’s not to discredit them. Like, you know, Amphiil does a lot of consulting and we have some amazing consultants, but, but they’re not all statisticians, so they just don’t get all that. So providing some routine data education for them, help them with their job, help them understand what you’re doing and they’re more likely to trust it when they do it. So I will say there is a pretty good level of healthy skepticism. So it is good that people are questioning things. So as data folks, we shouldn’t get upset if people are questioning what we’re doing. ’cause That’s in the end what we’re also doing and we’re asking questions and wanting answers to to get that. So informed confidence, I think is your probably your best goal. You want people to be confident in the work that you’re doing and why, know why they’re confident in it. So those are just a couple of things that I like to encourage folks to do to build that trust.
Glenn Hopper:
As you were talking about that, I was thinking about, there are so many drag and drop ML tools out there that do really cool stuff. And I was at a company years ago that actually had access to DataRobot, which is an amazing, I don’t know if you’ve ever played around with it, but a real, it’s the, the coolest, or it was, it’s, it’s been several years, but back then it was the coolest drag and drop machine learning platform I’d ever seen. And so you could have all these people who had no idea anything with, with data. We, we were just dropping in data sets and, and having the, the application pick the model to use. And they’re saying, look at this, we’re gonna do this, we’re gonna do this. And then, but the trust and data is, I, I have no, I, that was a K means clustering.
I don’t know what that is, <laugh>. So it’s, you know, you have to be careful with it too. And I think everywhere from Power automate to, you know, all these other drag and drop tools out there, you do have to have that domain expertise. And I think generative AI is gonna make these tools accessible to a lot of people who never had access to ’em before. But if you don’t understand what you’re modeling and you don’t really understand the data, or maybe you’re not even all, you know, you have different sources of truth, you don’t, don’t have a single one identified. I mean, there’s all kinds of pitfalls around this. And I think to really get that trust in the data, you’ve gotta build that culture. And that doesn’t just come from the finance group that has to be company-wide, but it is you know, I understand why people are skeptical and there’s that George Box quote that I use all the time that, you know, all models are wrong, some are useful, and we’re just trying to be as useful as possible with the stuff. <Laugh>.
Yeah, we’ve been talking kind of at the very high, sort of professorial level on all this, but I know you’re out there solving problems every day. And one of the things we talked about before the show was you did a, a basically a simple analysis that you did on on lost sales opportunities recently. Can you walk us, I want, I want, I wanna give like some more, like a more practical hands-on idea of, of some of the stuff we’re talking about. So could you walk us through how you went beyond just reporting the numbers to actually telling the story behind the data with that?
Skip Kastroll:
Sure. I had a really fun time with this one because I wanted to take a simple request and simple math essentially, and turn it into something that was a bit more compelling. So the base behind it is that we have sales opportunities, and if you lose a sales opportunity, you have a dropdown for a list of nine reasons for why you lost that, that sales opportunity those were previously agreed upon at the firm and what they mean. And so most folks at the organization know what they mean. So then I just took all of our closed loss opportunities over a period of time, and then I wanted to know like, what, what is this telling us? And so what about the organization? What about the person who made the sale? And just all the information that is typically cataloged when you have an opportunity in your pipeline.
So the math behind it was disturbingly simple. I mean, we’re talking percentages like that. There is, it’s hard to call it math. I mean it is math, but it’s hard to call statistics. But so it was pretty, pretty simple. So I could have just on like a one pager and said, these are the most common reasons, and this is a timeframe. And kind of left it up, could have done it in literally one slide, but I wanted to make it a bit more compelling, memorable, and have folks listen to it. So I created a port a report and I gave it a catchy title to kind of grab attention. So I titled it Unlocking the Secrets of Lost Sales Opportunities. So kind of almost immediately communicates that there’s value in this analysis. I also put a fun quote at the bottom that I’ve used in a couple different places, and the quote was data is the new oil, but we keep losing the map to the oil field.
So kind of like, you know, it’s important and it’s a challenge, but it is a little catchy. So a nice catchy title that was at the beginning like in like almost, I don’t wanna say inspiring, but a memorable quote there. I then gave a summary right away. Like, the very first thing was I, I gave a, a summary of the entire report. The report was like nine or 10 pages long, and I gave a summary at the front. Some people want to read the whole report and some people are gonna stop on page two. So I gave a quick summary. I use things like groundbreaking study, dive deep and prepare to be enlightened. Maybe a little over the top, but people are still talking about it. I got a question about it earlier in the week. So it was, it worked there.
I then, then took the distilled key findings into three main takeaways. So instead of just presenting the data, I provided what my best interpretation of the data was. So I really wanted the, our executives to kind of really quickly grasp the most important point without necessarily having to read the whole report. And then each takeaway was actionable. And, you know, it kind of linked the data back to a potential strategy. So, you know, we lost this, this was our most common lost opportunities, and this is what this might mean, and these are some strategies that we might be able to employ to combat this. So each one of those, so I gave three main takeaways. I then had, you know, a bunch of detailed analysis below for each finding. I give some context and implications. I also added, you know, great visuals using my my reference chart and things that people could remember.
I also tried my best to almost personify the data. Instead of just presenting numbers, you know, I kind of framed it as revealing secrets, uncovering mysteries, telling us a story. Again, may maybe a little over the top, but it’s helped folks remember it. Nobody groaned, nobody got upset at me. They enjoyed talking through this. I also got since there were different types of folks that were gonna be in the meeting or that were gonna read this report, I tried to tailor different sections to specifically different types of people. So this report went to the CEO, but it also went to individual directors who lead a team. And so I broke out some of the numbers by teams, by groups so that they would have something relevant that they could look at. And then kind of reiterated some potential key points from that.
So, you know, this group had the most lost opportunities for this reason, which might imply this, whereas this group had a different reason, which might imply something else. So there were takeaways for all sorts of folks that were gonna be quote in the room. So it was fun to kind of make this come like, you know, narrative structure over a, you know, potentially boring math report. Some really good visualizations and actionable insights. Some of my insights were way off base. Some of the, you know, as they looked at it, they were like, well, skip, that’s interesting, but you know, given what we know about what happened, this is probably more re likely what happened. And that’s great. That’s why they’re at the level where they are. They can see bigger picture, they can zoom out and they can see that.
And some of my suggestions that I provided were not necessarily as reasonable as they could be, but it kind of sparked some thought where they would think, you know, that, you know, we probably couldn’t do exactly what Skip suggested, but we could maybe do something like it. And so you can provide information there. And again, you’re positioning yourself as a partner. You’re someone who’s helping the rest of the team understand and interpret the information to become better, to advance whatever mission you have for where you’re working. So it was, it was a really fun report to make and you know, people are still ask about it some in jest, but they, they’re still engaging with it. So it’s, it’s, it’s been great.
Glenn Hopper:
Okay. And what I love about that story is, you know, you start with the data and maybe the data challenge wasn’t that much, but it’s, as more and more of what we do is automated, there is that component that for now anyway, is, is still the human element and also makes you a more valuable employee and a more valuable partner in the business is being able to take that data and turn it into that value add of, of recommendations and strategy. Whereas if you’re just spending all your time building the report with the data and everything, that is likely gonna be automated, you know, whatever level it is is likely gonna be automated in the next five years or so what are we gonna do to keep adding that layer of value there? So I I, I love that story and that example. So I’ve got a million questions, but we are, you know, we’re, we’re gonna be bumping up at time and I’m not gonna be able to, to get to all of ’em, I don’t think.
So I’ve got three that I wanted to ask, and I’m sort of gonna make an amalgamation of all three of these questions and, and throw it at you all at once <laugh> and see how it goes. But we, you know, we, we talk a lot about how for finance people is you know, be very good at fp and a, but I don’t consider myself a data person. And you know, and, and maybe I’m very good in Excel, but I’m, I don’t, I’m not gonna go become a computer developer and go learn Python. Maybe I can write SQL queries and that’s great. And that’s a part of my job and it’s, it gives me some value, but there’s so much out there right now where in Incomp and in a lot of companies, you’re the fp and a group, you’re not asked to be the data scientist or the, or the bi a person because you can get that information from them and you can use it in your reports.
But I’m, I’m really seeing to my mind, if you want to increase your value as a finance person, you need to, or you don’t <laugh>, I don’t wanna say you need to because there’s, there’s plenty of, of value that we add as finance people too. But if you really wanna stand out in the field, I’m thinking of some, some other people I’ve talked to recently who’ve really, they dive in and they’re, they’re doing Python and they’re, you know, now python’s integrated into Excel. So it’s just sort of an, an add-on feature. But seeing where we are now, seeing the ever increasing, it seems, I don’t know where it’s gonna end, but the ever increasing importance of data, if I’m, if my education I’ve got a master’s degree in, in finance and I’ve been doing fp and a for 10 or 15 years and I’ve been doing it in this environment, I mean, what advice would you have for them kind of looking ahead at where people are now, what’s gonna be important in the future, and how they could move from just thinking about everything in an Excel world to this kind of new world where the expectation, where the value that we’re gonna get is being masters of this data.
Like, I don’t know, that’s a lot to throw at you, but it’s, it’s sort of what’s a roadmap if somebody’s listening to this show right now and saying, okay, I see the writing on the wall, I need to get these skills. Where do they go from here?
Skip Kastroll:
Sure. I think one of the most important things that folks could do is kind of foster their own curiosity, which is a little abstract, but the question that I found that is most often in folks’ mind when they look at data and when you’re presenting it is, is the question of why. Like, why is this number the way it is? And so if you can start asking yourself that question as you’re looking at the reports that you’ve got, the data that you have and fostering that like, well, why do I think this is this way? That will then lead you into some more specific data, e questions, and then kind of, you’ll kind of frame your journey there. I think as far as data folks but I think this concept of data storytelling with visuals is just gonna keep growing. It’s gonna be more than just creating like a 30 page report, not that those aren’t valuable.
The, the historical context where the data came from, what it looks like is valuable, but the ability to tell the story of that 30 page report in a way that’s memorable is, is gonna be extremely helpful. How do you communicate those? I think professionals are eventually gonna need to be what I call bilingual, not in terms of like spoken languages, but the ability to speak data and also speak business and be able to interpret those back and forth. So not only do you need to understand the data behind it and understand the, the context of your business, but you need to be able to interpret back and forth to those, so understand the methodologies, but also understand how to clearly explain those methodologies in as simple of terms that exist. I think also more and more data folks or non-data folks are gonna be relied upon to do more than just analyze data.
They’re gonna be asked or expected to interpret that data. So more and more it’s gonna be like, you know, this is, we’re gonna not, and I’m being asked this regularly, like, it’s not just like, what is the data they’re saying, what does the data mean? What is it telling me? And as folks that understand statistics and analysis, there are some usually pretty clear implications from the data that we can share with relatively high levels of confidence and we should that doesn’t mean that we can do everything that is suggested but, you know, we can say like, sales are down 5% and what does this, why does this mean? And what, what, what is it? What’s going into this? And is there anything happening in the larger context? And so being able to frame that and interpret the data and provide strategies if it’s within your realm of expertise.
But, you know, we often, like, you know, I think we’ve like the quintessential like data person I often think of as the accountant, you know, money and money out and but they still have access to loads of information and they’re looking at these numbers day in and day out. So it’s often helpful to ask them, like, what do you see? What’s, what’s a trend that you’ve noticed in your daily work that just seems off? And they’re gonna have answers to that too. And so regardless of where you are in your data journey I think you can ask those questions of why, and then learn how to tell the story of the answer that you’ve got. This is in addition to understanding gen ai, like, you know, data manipulation, data analysis ETL kind of things, data governance. There’s lots of other, you know, really specific things that you certainly can learn. But I think the foundation of curiosity and being able to share the story are gonna be pretty, pretty paramount in the, in the years to come.
Glenn Hopper:
Yeah. Great insights. And you, well, you mentioned generative ai, and so that’s always gonna get me to <laugh>, get me to, to follow that up with a question. You know, and I I like to ask this of people like yourself who’ve been working with machine learning for years, and so, you know, the rest of the world is jumping on the AI bandwagon now, and you’re like, yeah, I’ve been telling you this for a while, <laugh>, but, you know but even people who are traditional ai, you know, machine learning folks and, and data analysts and machine learning engineers and all that, I mean, there are benefits to all of us, I think that can come out of generative ai. And I know that there’s, you know, there’s sort of challenges and opportunities around it. But when we talk before the show, we talked a little bit about how yes, there’s the traditional machine learning and, and that is, is tried, tested, and, you know, versus generative AI that’s still in, it’s you know, sort of the AOL early days of dialup phase, but there are applications for it. And I know you’ve played around generative AI a lot yourself. From your standpoint, what opportunities do you see in finance where we, you know, hallucinations a big problem? I mean, I, I guess in a lot of fields, hallucinations a big problem, but, you know, just the early phases, some of the promise that you’re seeing out of it. How do you see finance people being able to harness generative AI in the future?
Skip Kastroll:
I got a co several things that I often do in Gen AI and then I also recheck it because you mentioned sometimes it’s not perfect, but I think it’s gonna be like truly transformative. And I, I don’t really say that like flippantly. I think it really is. It, it’s providing access to like tools that otherwise just didn’t exist. Like, you know, you, you needed to know machine learning and how to build those models before you could do that. Now in Gen ai, you can dump in a data set and you can say, what can you find out about this data that you might understand? Now, again, to your point, you do need to double check that some things, gen AI is gonna do weird things. For example, if folks want to have a quick chuckle, they should go into Gen AI sometime today and ask Gen ai, how many Rs are in the letter strawberry?
Just see what it tells you. It, it’ll be fun. I’m not gonna spoil it for you. Just let them look that up. So Genai is gonna do some weird things, but it is still really powerful. I use it for data analysis assistance. Sometimes I’ll punch in some data and or a data table and I’ll have it turn some numbers out for me. Sometimes I’ll do that as a spot check for some work that I’ve already done, or sometimes I’ll do it the other way, I’ll put it in there and then I’ll check the back end of it so I can, you know, have done my homework, so to speak. I use it a lot for report generation. I’ll provide it with some information. I’ll say these are my, this is tables of data that I collected within this context. I’m presenting a report to the executive management team.
The report is gonna be 15 minutes long. Help me generate some key information from these tables and it will give you a ton of stuff. And then you can pick and choose what you think is valuable and great. So that’s really good. There as well data storytelling, if you struggle with telling the story of your data, I think gen AI can really, really, really help with this help. It is looking at like, the almost entirety of stop stories that are out there. Like you, you can pull it up if you want to ask Gen AI to say, make my report formatted, like Cormack McCarthy, it, it’ll do that for you. It’ll be hilarious and probably interesting, but it’ll still do that for you. So but you can put in simple data, specify your audience, specify the tone, and it’s gonna help you frame the data into like a more impactful story.
And then if you want, you can then change it into, so it sounds more like you, like I I use Gen AI and then I’ll, I’ll tweak it a little bit afterwards. ’cause I wanted to have like, you know, the skip to it and not, you know, not the gen AI to it. So it’s really helpful there. It can help with a lot of automations. So automatic routine financial processes, I think it’s gonna be a big thing that it helps with most of the tools now out there. Have some version of some AI in them that helps automate things. And so understanding what they are and how using and leveraging those tools will free you up to do things that it can’t. And so people often get nervous like, well, is this tool, is this thing, is Walmart gonna take my job?
But if you reframe it and think, how can I use this tool to make the parts of my job that are a little boring and that I could do a little faster, that will then free me up to do more valuable things, I think that that, that freedom can be helpful. It can be also be a little, you know, nervous to go through there. So, so I think gen I is gonna be super helpful. There’s loads of, you know, guardrails and guidelines you need to think about with ai. And there’s, if that’s a whole other conversation, but initially using it and helping with storytelling report generation, it’s, it’s, it’s really, really powerful. It’s made some really great PowerPoint decks for me. It’s also made some pretty terrible ones. But it’s made more helpful ones than more not helpful ones.
Glenn Hopper:
We’ve hit the time of the show where we this, we ask every guest this question and you know, it’s funny because I would think, oh, you’re doing all this machine learning stuff, you probably don’t spend that much time in Excel, but I kind of bet you do still spend a fair amount of time in Excel. So one of the questions that we always ask everyone is what is your favorite Excel function? And why?
Skip Kastroll:
Oh man, I got really excited about this <laugh>. So my favorite Excel function is using a combination of X Lookup with Index match. So I love pivot tables and pivot tables are near and dear to my heart. But I don’t often like the way Excel does calculated fields or calculated values in pivot tables. So you can use X lookup or vlookup normally, but they don’t follow your table. So if you expand your pivot table, your X lookup doesn’t copy down. But if you use X lookup with a combination of index match, it becomes this dynamic and powerful like add-on to your existing pivot table. So that’s truly one of my favorite Excel functions is x lookup com combined with index match.
Glenn Hopper:
Love it. Love it. Skip really really appreciated your insights and coming on, and I know I’ve, I’ve just been en enjoying having data folks like yourself on, and, and hopefully it’s been informative for our audience. And I guess you know, before you go what’s a good way for people to get in touch with you?
Skip Kastroll:
Probably the easiest way is LinkedIn, unsurprisingly, but you can find my profile like slash Skip Castro and then it’ll probably be in the show notes. If you wanna just make a note mentioning that you heard me on FP&A podcast, so we can chat about a couple of things. So that’s probably the best way to connect with me. You can also email me skip@amphil.com. It’s a pretty simple one. Always eager to talk about what data you’re using, how you think it can be done better, or obviously we can chat about cycling ad nauseum. So, so those are two ways. So really thankful to be on here. I really, really enjoyed this conversation. My, you know, journey through math and education is, has brought me to a pretty, a fun place of interacting with data and storytelling. So it’s, it’s, it’s really fun and I’m really excited to, to continue doing this and the work that I do and then helping others do it well too.
Glenn Hopper:
Well, really appreciated your time and insights.
Skip Kastroll:
My, my appreciate it. Glenn. Anytime you wanna talk about fantasy fiction or if you wanna talk about data you just gimme a call. <Laugh>.
Glenn Hopper:
Alright, that’s a deal. That’s a deal. <Laugh>.