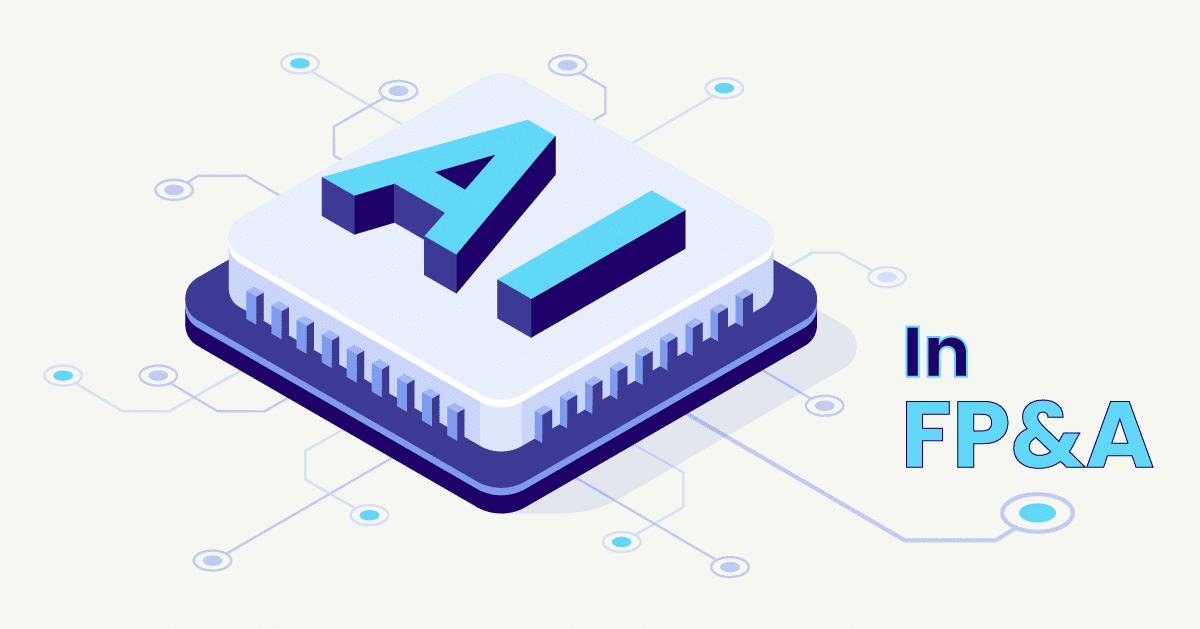
AI is a hot topic. It’s also a buzzword that is used in a lot of different ways. Will AI revolutionize the way we work? Will AI replace our jobs? How will AI affect finance and FP&A in particular? These are just some of the questions that finance professionals are asking themselves today.
According to an FP&A Trends survey from 2021, 11% of organizations make use of AI or Machine Learning (ML), while 57% of organizations are planning to use it in the next few years.
Keep in mind those numbers are pre-ChatGPT and AI hype.
According to a more recent NVIDIA report, 91% of financial service companies are either assessing AI or actively using it for things such as “driving innovation, improving operational efficiency and enhancing customer experiences”.
What is AI in FP&A?
AI is used in a lot of different contexts and industries. While AI is not a new technology, it has grown exponentially in the past few years. An even more important occurrence was the release of ChatGPT and other chatbot functions that opened AI to the general public.
This allowed people with almost no tech experience to benefit from AI. ChatGPT became the fastest growing product of all time by reaching 100 million users in less than 2 months!
To put it into perspective it took Facebook more than four and a half years to reach 100 million users.
It is evident that AI will change the way we do many jobs.
The same is true with AI in finance. AI has the potential to revolutionize and disrupt the way FP&A professionals and finance teams work. In fact there are already many finance AI trends in 2024 that are reshaping the way finance teams work.
Instead of spending so much time on manual processes, finance can focus more on high-value tasks and strategic decision making. While financial automation software already exists in many different forms, AI has the potential to automate the repetitive work that computers know so well, and even get faster and more accurate as time goes on.
A more advanced way that AI can change FP&A and finance is through a chatbot function. Chatbots can help automate repetitive tasks, but more importantly they can pull data and answers to questions quickly. In this case, a finance Chatbot would be similar to ChatGPT, with the differences being that a finance chatbot needs to be more accurate, up-to-date, and secure.
There are quite a few finance software companies that are releasing an AI chatbot like this, and it has the potential to greatly improve the speed and collaboration between finance and management.
The most advanced function of FP&A AI is predictive analytics. In this case, a user can plug in data and receive a whole dashboard of information about what is the most likely occurrence based on past history, the current market, and many other factors. While predictive analytics exist to some degree today, it is still quite far from its ultimate function where AI can pretty much do everything that FP&A professionals do, and much faster.
Predictive analytics brings up the question of whether AI will replace FP&A jobs. Skip to the section that talks about whether AI will replace finance jobs to learn more.
Difference between AI, Machine Learning, and Predictive Analytics
AI, machine learning, and predictive analytics are closely related concepts and are often used interchangeably. However, they have distinct differences, especially on the topic of finance and FP&A.
Artificial Intelligence (AI)
AI is a broad field that aims to develop intelligent machines capable of simulating human intelligence and performing tasks that typically require human intelligence. It encompasses a range of techniques and approaches to create systems that can perceive, reason, learn, and make decisions. AI can be further categorized into two types:
a. Narrow AI- Also known as weak AI, narrow AI refers to AI systems designed to perform specific tasks or solve specific problems. These systems are highly specialized and focused on a particular domain.
b. General AI- Also referred to as strong AI or AGI (Artificial General Intelligence), general AI aims to create machines that possess human-like intelligence and can perform any intellectual task that a human can do.
Machine Learning (ML)
Machine learning is a subset of AI that focuses on the development of algorithms and models that enable computers to learn from data and improve their performance without being explicitly programmed. ML algorithms can analyze large datasets, identify patterns, and make predictions or decisions based on the data.
ML can be categorized into three main types:
a. Supervised Learning- In supervised learning, the ML algorithm is trained on labeled data, where each data point has a corresponding target or label. The algorithm learns from the labeled examples to make predictions or classify new, unseen data.
b. Unsupervised Learning- Unsupervised learning involves training ML algorithms on unlabeled data. The algorithm learns patterns, structures, or relationships within the data without explicit guidance. It aims to discover hidden insights or groupings in the data.
c. Reinforcement Learning- Reinforcement learning involves training an agent to interact with an environment and learn from the feedback it receives. The agent takes actions in the environment to maximize a reward signal, learning optimal strategies through trial and error.
Predictive Analytics
Predictive analytics refers to the use of statistical techniques, data mining, and machine learning algorithms to analyze historical and current data and make predictions about future outcomes or behaviors. It aims to uncover patterns, relationships, and trends in data to forecast future events or behavior.
Predictive analytics often utilizes ML algorithms to analyze large datasets and identify patterns that can be used for making predictions. It involves using historical data to train models and then applying those models to new, unseen data to generate predictions or insights.
To sum it up, AI is a broad field concerned with creating intelligent systems, while machine learning is a subset of AI that focuses on enabling machines to learn from data. Predictive analytics, on the other hand, uses statistical techniques and ML algorithms to make predictions based on historical and current data. ML is a key component of predictive analytics, as it provides the algorithms and techniques to analyze data and make predictions.
Benefits of FP&A AI
1) Make decisions and scenario plan in real time
One of the biggest problems in the finance department is not being able to act quickly enough. So often, the data that is being analyzed is from last week, month, or quarter. If companies had financial AI capabilities and were able to see all of the data in real time (and on top of that scenario plan in real time) it could help tremendously in taking advantage of opportunities that need to be acted on quickly. This would also give them a big advantage over competitors as they would be the first ones.
2) Cut down on employee costs and manpower
A big advantage of AI and automation is cutting down on costs. In many industries, labor costs (salaries, benefits, etc.) are the biggest expense, and can make up to 70% of all the company’s expenses. Naturally, looking to cut labor costs is a great way to increase profit margins.
AI can do just that. Chatbots and predictive analytics can help reduce the finance team by replacing much of the repetitive and manual work that in the past had to be done by a usually well-paid finance professional.
3) Leave more time for analysis and strategic thinking
Even if you have a small finance team or are not looking to cut down on employee costs, AI for FP&A will free up a lot of time for more analysis and strategic thinking. Studies show that even with all of the automation tools available today, finance teams can spend well over 50% of their time on manual tasks. Imagine if that time was spent on strategic planning and business partnering instead?
4) Reducing human error
One of the biggest advantages that humans have over software is the emotional and human elements. Machines can’t connect to humans in the same way (at least not yet). But human work is also a cause for error. Finance teams have made some expensive and comical errors in the past, and the more manual work there is, the more chances for data sets and reports to contain mistakes.
While it is important to have humans in finance for things like storytelling, reporting, problem solving, and going over reports, the combination of AI/ automation and human employees is ideal.
AI Challenges in FP&A
1) Still has a ways to go
Quite simply, AI is not yet ready to be a mainstream tool in the finance department. While financial software companies are working on developing specifically AI based finance tools, FP&A AI still has a ways to go until it is commonly available for all types and sizes of companies.
2) Data Integrity and Security
One of the biggest problems with AI to date, especially for finance, is that the data is not trusted and secured. Companies can’t rely on the platform, as there hasn’t been enough measures taken to secure it. There is a lot of talk about future government compliance laws in regards to who can use AI and for what, and that talk brings uncertainty. Public and private companies alike are concerned about this, and until there is more trust in the security of the data, it might be a hindrance to financial AI.
3) AI doesn’t solve everything
No matter how advanced AI becomes, finance professionals will still be needed to run it, report the data, and take the correct actions. It might help cut down on the amount of time and human resources needed in finance, but for now, FP&A is not going anywhere. That being said, AI is not a quick fix, rather a tool that can greatly improve the finance department. Collaboration, storytelling, and strategic decision making are still aspects that will need to be run by humans. Companies will still need to invest in their FP&A team, and with the finance and accounting shortage that exists today, it’s not always so easy.
Future of AI in FP&A
AI has the potential to completely disrupt the job market, and finance is no different. The future of AI in FP&A holds immense potential. Key aspects include increased automation, advanced predictive analytics, real-time insights, natural language processing, enhanced visualization and reporting, and improved risk management. The question isn’t whether AI will disrupt FP&A, rather when and how much.
The most immediate disruption will be ChatGPT-style FP&A chatbots. In fact, they are already here. Some FP&A solutions, like Datarails, already announced their development of AI powered chatbots, and this has the potential to provide trusted and real time answers.
But human professionals will continue to play a crucial role in interpreting results, making strategic decisions, and ensuring ethical considerations are met.
Will AI Replace Finance Jobs?
“Will AI take my job?” is usually one of the first questions that people think of when AI comes to their industry, and rightfully so. Most sci-fi movies involving AI don’t end so well..
But similar to all technological advancements throughout history, industries will be changed, rather than erased completely. For those who lead the change and learn how to use it, there are immense opportunities.
AI excels at automating repetitive tasks, analyzing large volumes of data, and providing insights. This can free up finance professionals from routine activities, allowing them to focus on higher-value tasks that require human judgment, strategic thinking, and decision-making. Finance professionals will still be needed to interpret and communicate AI-generated insights, ensure compliance and ethical considerations, manage relationships with stakeholders, and provide strategic guidance based on their expertise.
Moreover, the finance industry requires trust, accountability, and regulatory compliance, which necessitate human oversight. The complexity of financial decision-making and the need for contextual understanding and critical thinking further highlight the value of human professionals in finance, especially in the early stages of AI.
However, there are a few things to keep in mind in regards to how AI will change finance careers.
- For big finance teams there is a possibility that headcount will be reduced. Although a company will still need employees for analysis, compliance, and collaboration, it might be possible to reduce headcount if AI can cut down on the amount of manual work.
- It might be harder to get started in a finance career. Most people start their finance careers in positions involving a lot of manual work, such as pulling and consolidating data, or junior analysis roles. AI can automate most of this “starter” work and companies will look more and more for finance professionals who come with experience and tech knowledge. This could be a big hindrance for people starting their finance careers who generally rely on manual roles to get their foot in the door.
- There will be a bigger emphasis on those who keep up with technology. Although many AI tools are quite simple to use, there are still best practices and constantly emerging new tools and ways to automate. Companies might prefer finance professionals who can keep up with these trends over those who don’t.
While certain roles within finance may evolve or change due to automation and AI integration, new roles and opportunities are likely to emerge. The successful integration of AI in finance will require a combination of technical skills and financial expertise, allowing finance professionals to collaborate effectively with AI systems.
Examples of Companies Using AI in their FP&A Processes
Egencia
Egencia, the travel management company used by Expedia, started using AI back in 2019 to improve its forecasting processes.
Egencia had trouble with its forecasting, namely that it wasn’t so accurate. The company needed it to be better both for their own benefit and for all of their business partners who were struggling with inaccurate forecasting in such an important field as travel.
The company started using Machine Learning for developing a driver based P&L forecast based on the previous 4 years of historical data. What used to be done by 100 people was then completed by just the forecasting tool and became faster, far more accurate, and lowered the refresh times.
Microsoft
Takeshi Murakami, Group Controller at Microsoft described to news site FP&A Trends two ways that Microsoft is using AI in their finance team:
- “ML enables us to learn from the past to predict the future, we can know WHAT WILL HAPPEN. The distance between DATA and ACTION is now shorter , the amount of Manual processes and human errors is lower. In the past in Japan forecast preparation, roughly 60 employees plus management and stakeholders worked on quarterly forecast spending 2 – 3 weeks, plus a couple of executive reviews. But with this model today, we can run it with just 1 or 2 employees and it is in almost real time because the ML is linked directly to our database. And with all the efforts Microsoft Corporate and data scientists spent on adjusting the model and improving the accuracy, ML forecasting model is now more accurate than the number created by humans. Still, we run both in parallel, but gradually shifting to the ML model. The key message here is not only the accuracy but also freeing up all those employees involved. Now they can shift their time on more value-added work and drive executions.”
- RECOMMENDATION. “Finally with a combination of Machine Learning and Bots we get to the position that we can say WHAT TO DO, automating the decision making process and moving really fast from DATA to DECISION. One of the examples is Compliance predictive analytics where AI/ML will flag the unusual sales transaction in the system and raise an alert, then notify the finance controller to check the background of the deal, discount given, partners involved etc. So we are at the stage now where the Machine tells controllers what to do, this immediately leads us to take action.”
Conclusion
Like so many industries, AI will disrupt how the finance department works as well. It’s not a question of if, rather it’s a question of when and how much. AI chatbots are already here and they will help reduce manual work and get answers quickly. In the future, predictive analytics will reduce manual work even further by doing consolidation and analytics in real time.
While certain roles within finance may evolve or change due to automation and AI integration, new roles and opportunities are likely to emerge as well. The successful integration of AI in finance will require a combination of technical skills and financial expertise, allowing finance professionals to collaborate effectively with AI systems.