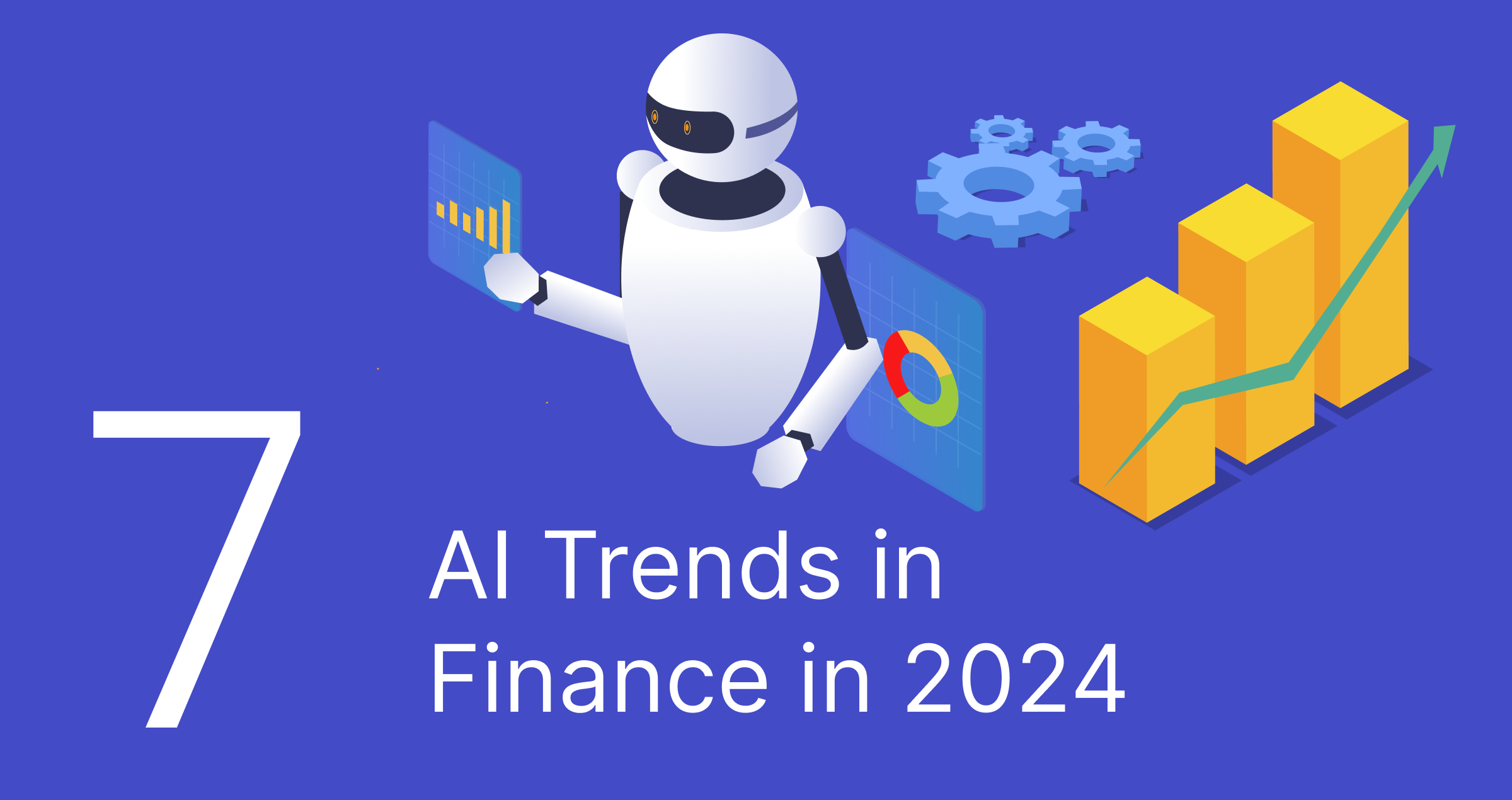
Artificial intelligence is a hot topic of conversation in virtually any industry. However, AI is proving to be particularly transformative in finance and FP&A.
The financial landscape in 2024 is buzzing with AI-driven initiatives aimed at enhancing efficiency, security, and user experience.
Here, we have amassed seven crucial AI trends shaping finance’s future.
7 AI Trends in Finance in 2024
From personalization to the surging field of decentralized finance, each trend underscores AI’s seismic impact on financial services.
1) AI-Driven Personalization
Financial entities use sophisticated algorithms that analyze customer behaviors, spending patterns, and economic objectives to offer tailor-made advice and products.
Here are just a few examples of how AI-driven personalization in finance works in practice:
- Data Analysis
Financial institutions collect and aggregate customer data, including transaction history, online behavior, social media activity, demographics, and financial goals.
- AI Algorithms
Advanced AI algorithms, such as machine learning and deep learning models, are deployed to analyze this data and identify patterns, trends, and correlations that can provide insights into individual customer preferences and needs.
- Personalized Recommendations
Based on customer data analysis, AI algorithms generate personalized financial product and service recommendations. This could include investment strategies, savings plans, insurance policies, credit products, etc.
- Dynamic Adjustments
AI-driven personalization is a process that takes time. These algorithms keep learning and adapting to new data inputs and customer interactions. This allows financial institutions to better refine their recommendations over time to suit each customer’s evolving needs and preferences.
- Improved Customer Engagement
By offering personalized recommendations and experiences, financial institutions can enhance customer engagement and loyalty. Customers are more likely to trust and value services tailored to their specific circumstances, increasing satisfaction and retention.
- Risk Management
AI-driven personalization also extends to risk management, where algorithms assess individual risk profiles and behaviors to customize risk mitigation strategies, such as fraud detection, credit scoring, and portfolio diversification.
2) RegTech Advancements
Regulatory compliance, the thing that has long plagued financial institutions, is now being tamed by AI. Tasks that traditionally consume tons of time and resources are streamlined through automation. AI-driven regulatory technologies are realizing real-time risk assessments, expeditious compliance reporting, and even proactive fraud prevention.
Bank of America’s virtual assistant ‘Erica’ is one example. It streamlines customer interaction with regulatory norms, thereby mitigating compliance risks.
This is part of a broader trend of large financial institutions being more willing to invest in AI. According to the International Monetary Fund, financial institutions are set to double their spending on AI by 2027.
For finance teams, AI-powered document analysis solutions can quickly scan through volumes of text to identify and interpret relevant information. This vastly improves compliance with regulatory requirements and reduces the risk of human error and costly penalties.
3) AI-Powered Forecasting and Budgeting
AI-powered forecasting and budgeting revolutionize financial planning by harnessing advanced algorithms to analyze extensive datasets. These datasets encompass historical financial records, market trends, economic indicators, and other relevant factors. Through this comprehensive analysis, AI facilitates the generation of highly accurate projections. It allows finance teams to make more strategic decisions and allocate resources more effectively.
Unlike traditional static models, AI-driven systems offer dynamic modeling capabilities. These allow for real-time adjustments based on evolving data inputs and feedback. This adaptability enables finance teams to respond swiftly to changing market dynamics and unforeseen events, allowing forecasts and budgets to remain relevant and reliable.
AI also facilitates scenario analysis and risk assessment by simulating different future scenarios and their potential impacts on financial performance. Organizations can then identify risks, opportunities, and alternative strategies to mitigate threats and capitalize on opportunities.
Finally, AI-powered forecasting and budgeting streamline the planning process, reducing manual efforts and freeing time for strategic analysis and decision-making. AI systems’ iterative learning process further enhances accuracy and reliability over time, providing ongoing value to finance teams and the organization as a whole.
4) Dynamic Modeling and Adaptation
We’ve mentioned how AI-driven systems offer dynamic modeling capabilities that allow real-time adjustments based on evolving data inputs and feedback. Let’s dive deeper into this aspect of AI in finance.
Dynamic modeling refers to the ability of AI systems to continuously adjust and improve forecasts and budgets based on changes in market conditions, consumer behavior, and other relevant factors. This is possible thanks to advanced algorithms that analyze large volumes of data in real time and make predictions with a high degree of accuracy.
This adaptability is crucial for finance teams—it allows them to respond quickly to fluctuating market dynamics and unforeseen events. For example, during the COVID-19 pandemic, traditional financial forecasting methods may have been rendered obsolete due to the unprecedented nature of the crisis. However, AI-powered systems were able to quickly adapt and provide accurate insights and predictions, helping organizations make informed decisions in a highly volatile environment.
Furthermore, with the help of AI, finance teams can efficiently run various scenario analyses to evaluate the potential impact of different business strategies and external factors on financial performance. This allows for more agile decision-making and risk management, ultimately improving economic outcomes.
5) Predictive Risk Analytics
AI’s predictive capabilities are wielding a revolutionary influence over risk management. Thanks to machine learning models, financial forecasts, once ridden with uncertainties, are now endowed with precision and foresight. These work by analyzing massive amounts of data to uncover hidden patterns, anomalies, and risks that may undermine a company’s financial stability.
AI-powered risk analytics can also help finance teams identify potential fraud or unethical practices within the organization. This helps them proactively mitigate these risks before they escalate into more serious problems. For example, AI can flag suspicious transactions or irregularities in financial reports, allowing finance teams to investigate and take necessary action.
6) AI-Powered Chat FP&A
Tools like Datarails’ FP&A Genius leverage AI to efficiently address vital inquiries concerning business budgets, forecasts, variances, and expenditures. Our innovative AI-driven chat service delivers insightful responses by analyzing comprehensive and unified finance data from various departments within the organization.
This helps solve one of the biggest problems that finance professionals experience: Having to sort through data and spreadsheets in order to answer a simple finance question.
By leveraging AI algorithms, FP&A Genius swiftly identifies patterns and trends in real time, allowing businesses to make informed decisions promptly.
7) Expense Management Automation
AI-driven expense management systems revolutionize how businesses handle finances by simplifying the task of tracking, categorizing, and reconciling expenses. With the help of advanced algorithms, these systems not only automate these processes but also have the capability to:
- Identify recurring patterns
- Detect anomalies
- Pinpoint potential cost-saving opportunities
This proactive approach enhances operational efficiency and minimizes errors, leading to better financial management practices.
Strategies for Utilizing AI Trends in Finance
As exciting as AI technology may seem, having a well-defined strategy before implementing any AI-powered solutions can mean the difference between a successful and unsuccessful integration.
Some strategies businesses can utilize to optimize AI trends in finance include:
Identify Business Objectives
Begin by defining clear business objectives and goals that AI-powered solutions should help achieve. Align AI initiatives with overarching business objectives, whether:
- Improving operational efficiency
- Enhancing customer experiences
- Reducing costs
- Mitigating risks
Data Quality and Accessibility
Ensure high-quality data is available for AI analysis. Invest in data infrastructure and governance practices to effectively collect, clean, and organize data from various sources. Accessibility to data is crucial for training AI models and generating valuable insights.
Start Small, Scale Gradually
Begin with pilot projects or proof-of-concept initiatives to demonstrate the value of AI solutions. Start with specific use cases or areas within finance where AI can deliver immediate benefits. Once successful, gradually scale up implementation across different functions and departments.
Collaboration Between Finance and IT Teams
Foster collaboration between finance and IT teams to ensure alignment of AI initiatives with technical capabilities and infrastructure requirements. Finance teams should communicate their needs and requirements effectively to IT teams responsible for implementing AI solutions.
Invest in Talent and Skills
Develop a workforce with the necessary skills and expertise in AI technologies, data analytics, and finance domain knowledge. Provide training and professional development opportunities to upskill existing employees and recruit top talent with AI expertise.
Ethical and Regulatory Compliance
Prioritize ethical considerations and regulatory compliance when implementing AI solutions in finance. Ensure transparency, fairness, and accountability in AI algorithms and decision-making processes. Prioritize compliance with relevant regulations and standards that govern:
- Data privacy
- Security
- Ethical AI practices
Regular Monitoring and Evaluation of AI Trends in Finance
Implement mechanisms for continuous monitoring and evaluation of AI-powered solutions. Regularly assess performance metrics, user feedback, and ROI to measure the efficacy and impact of AI initiatives. Iterate and refine AI models based on insights gained from monitoring and evaluation processes.
Customer-Centric Approach
Keep the end-users, whether internal stakeholders or external customers, at the forefront of AI initiatives. Design AI-powered solutions that enhance user experiences, address pain points and deliver value to customers. Solicit feedback from users and incorporate their input into the development and refinement of AI trends in finance.
Ready to Adopt These AI Trends in Finance?
Whether for global financial powerhouses or personal financial management, AI’s role is both pivotal and expanding. Be on the lookout for our forthcoming article describing precisely how AI is used in finance, which will explore a more hands-on approach detailing the practical implementation of AI within the financial sphere.
In the meantime, explore the AI-powered FP&A tools that Datarails has to offer and our suite of features designed to help finance teams spend more time analyzing data and less time collecting it.
Did you learn a lot about AI trends in finance from this article?
Here are three more to read next: