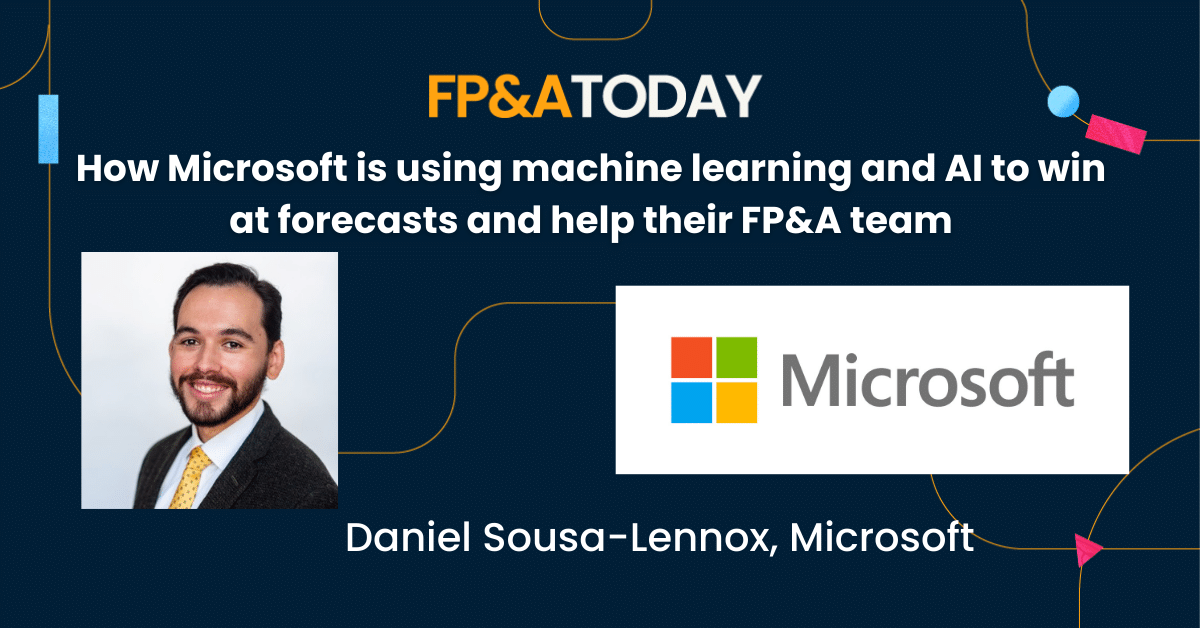
How is Microsoft using AI to reach up to 99% accuracy in some forecasts? In this episode of FP&A Today, Daniel Sousa-Lennox, a data scientist at Microsoft with deep experience of financial analysis, reveals all.
The Microsoft’s finance team – about 5000 people – saw its first foray into machine learning in forecasting. The team developed FINN (or Microsoft Finance Time Series Forecasting Framework) says Sousa-Lennox who sits within the finance organization, providing software engineering and data science expertise. Using machine learning and creating AI finance tools (such as FINN which has been open-sourced) Sousa-Lennox says the Microsoft finance team has replaced burdensome forecasting and Excel heavy lifting. “The tool has helped, for instance, this one team in terms of accuracy to get the revenue that they were trying to forecast for an Azure product (the cloud computing platform run by Microsoft) to 99% accuracy”. But Sousa-Lennox adds that it is important to remember that AI is not “magic” and “wont lead to 99% in every single forecast” and the quality of data is paramount.
Nevertheless the results are transforming financial forecasting at the $2trillion company.
FINN alone provides access to 25+ models supporting Microsoft’s daily, weekly, monthly, quarterly, and yearly forecasts, transforming the approach of FP&A.
Sousa-Lennox says: “It takes on average for a forecast used run using our tool, it takes about an hour to an hour and a half, which is significantly less than a week [previously]. It is also an hour and a half where you don’t have to be actively doing anything.”
In this episode Daniel Sousa-Lennox shares:
- Similarities and differences between FP&A in US and Panama (and banking vs Microsoft)
- Getting an internship to full-time career at Microsoft
- How he trained in Data science and technology
- How to evangelize finance teams into adopting AI
- How Microsoft is transforming its forecasting and FP&A
- Despite its success why AI is not a “magic bullet” for finance
- The two most powerful lessons in presenting AI to finance teams
- The biggest advice for anyone starting in FP&A
- Last thing finance thing I Googled ChatGPT’d
- My favorite Excel function
Links
Follow Daniel Sousa-Lennox: https://www.linkedin.com/in/dsousalennox/
FINN (Open-sourced by Microsoft): https://microsoft.github.io/finnts/
Paul Barnhurst:
Welcome to FP&A Today. I am your host, Paul Barnhurst, aka the FP&A Guy. FP&A Today is brought to you by Datarails, the best FP&A software for Excel users. Every week we welcome a leader from the world of financial planning and analysis. Today we’re delighted to be joined by Daniel Sousa-Lenox. Daniel, welcome to the show.
Daniel Sousa-Lennox:
Hi Paul. Thanks for having me. Super excited to be here.
Paul Barnhurst:
We are excited to have you here and for you to be here. And I just have to say, I know you’re in a good mood because you’re wearing a Texas shirt and for anyone who knows when this was recorded, Texas just beat Alabama, so I know Daniel’s happy.
Daniel Sousa-Lennox:
Yeah, very, very happy.
Paul Barnhurst:
So Daniel comes to us from the Seattle, Washington area. He currently works as a software engineer at Microsoft. He earned his bachelor’s degree from the University of Navarra in mechanical engineering and an MBA from the University of Texas. We’re going to start with, this is a question we like to ask everybody, a fun one. What is the most challenging budgeting forecasting experience you’ve had in your career?
Daniel Sousa-Lennox:
Yes, right after graduating from college, I started working at a bank back in Panama where I’m from and I was in the strategic planning department, so it was a mix between finance and strategy. So every three, four years, the bank goes through the strategic plan process, which is like a holistic overview of let’s see where we want to take the company for the next three years, what things we want to do that are different from what we’re doing. So I was in charge of a few of these new strategies or new strategic initiatives, but then I was also in charge of all the modeling, financial modeling of such initiatives. So what that meant was we had to model a budget as if business as usual were to remain. But then in parallel we had to model what if we bring in this new initiative, what would the impact be in all of our revenue cost, et cetera.
So that was probably the most challenging one because it was basically doing two separate budgeting processes at the same time and you have to keep track of them, make sure which ones is not taking into account the initiatives, which ones are, and then you had to be able to toggle on and off certain initiatives. Maybe you didn’t want to approve all of them, just a few. So it had to be a very dynamic spreadsheet, let’s say, so that if your leadership team came and they asked for, hey, I want to see initiatives two, four, and six, what’s the impact on our bottom line? So it had to be a very well made spreadsheet and all the concept or the ideas had to be very clear so that we could very quickly iterate and change what we needed to change. So it was very challenging from an operations perspective and then also from a people’s perspective, having to deal with, as a finance person, you don’t work alone, you have to partner with whatever product or service team you are budgeting for or helping budget for. So that was also challenging because since it’s the strategic plan, it’s basically partnering with everyone. So I think that’s for sure the most challenging process budgeting process I’ve been through.
Paul Barnhurst:
Anytime you got to run multiple versions, it can become really challenging. I had one year where halfway through the budget they decided we had to break it out into four distinct scenarios and they all had a layer on top of each other so we could show what each one looked like and turn ’em on and off and it was rough. So I can relate a little bit to that. What did you learn from that experience? What was your key takeaway?
Daniel Sousa-Lennox:
Yeah, I think I had two key takeaways. One from the operation side of building a budget and then one from the people dealing with people with stakeholders. Like on the operations side, I think it was whenever you’re going to embark on a big modeling project, take the time to build the model with intent and strategically knowing that things will change. Make everything you cannot account for everything that will happen, but try to in the way that no hard coding things, even if new requests come in middle of the modeling process, never assume that it’s going to be a one-off thing. It’s also going to change. So every time you incorporate a new thing, try to from the start, make it dynamic, easy to change, keep track of everything because at least in this one, I hit a few roadblocks or hiccups because of a last second request that I thought, oh, this is not going to change, so I’m just going to hardcode this real quick.
But then not even a day later, it was already changing and then I had to go back. So on the operation side of maintaining the spreadsheet, if that’s the tool you’re using, definitely taking the time of thinking through how is this going to be mutating as the process goes along. And then on the people side, it was probably the fact that whenever you’re creating a budget that someone else is the one that’s going to have to meet that number. There has to be absolute buy-in from them when you’re coming up with those numbers. So it has to be not only working with them, obviously going to happen, but making sure that they feel like the number came from them, even if it didn’t, but make them feel like it came from them so that they have basically buy-in on that number. And then that’s an easier sell for leadership. You have to sell that number to your finance leadership team, but then also to that team’s leadership team. And when their team is the one doing the selling, it’s way easier than when it’s the finance guy that’s coming to someone in marketing, whatever and telling them this is the number. Because I say, so I was the other key piece that I learned.
Paul Barnhurst:
Those are two fabulous takeaways, so I’m just going to comment on those both real quickly. The first one, design a hundred percent agree, design your model with intent. Think about it, make sure you have those inputs, calculations, outputs in such a way that it’s easy to update. You can make changes, roll things in. Now are you going to be able to anticipate everything like you said? No, never. Are you sometimes going to get caught with something that requires a ton of work? Yes, but definitely it will help prevent the hard coding that we talked about or the other things. I mean, I couldn’t emphasize design enough. That’s one of the courses. I have a digital course about design principles for modeling because I think it’s a real challenge. And then the second one, buy-in. I’ve been in meetings where I don’t recognize that number. Finance made it and I see you smiling. You never want to be in that situation, right? And I love how you said make them feel like they have ownership even if it wasn’t the number they wanted. You need to make sure you get that buy-in that they’re going to at least be committed to whatever decision was made.
You know what it is like 13 different spreadsheets emailed out to 23 different budget holders, multiple iterations, version control errors, back and forth updates you never really feel in control of the consolidation and collection process. Yep, I’ve been there. Stop. Breathe. E data rails is the financial planning and analysis platform for Excel users. Data rails takes data from all your company’s disparate sources. No organization is too complex, consolidating everything into one place, secured in the cloud now all your data finally talking to each other, everything is automated. Back into your report in Excel. Cashflow FX conversion, intercompany transactions now automated and UpToDate drill down and variance analysis in seconds. Don’t replace Excel, embrace Excel, turn your Excel into a lean mean fp and a machine. Find out more@www.data rails.com.
So can you take a few minutes and just tell us about yourself and your background? I know you started in Panama, you’ve came to the US and you have a great, great background. So just walk us through a little bit about that and how you ended up where you’re at today.
Daniel Sousa-Lennox:
Yes, yes. I’m from Panama. I went to college in Spain. So those four years I spent in Spain studying mechanical engineering. Then as soon as I graduated, I came back to Panama, but I never practiced anything related to the engineering field at the time. I immediately pivoted into finance and strategy in the banking industry. I did that for almost five years until I decided that I wanted to go to grad school, get an MBA. And since I had already been to Europe and now I wanted to get the experience in North America, I was between the US and Canada
And I ended up deciding to go to the University of Texas when I did my MBA. It was a general MBA, but with a little bit of a emphasis on business analytics. That was my first academic exposure to business analytics. Obviously in my job in finance, I had some through hard work, had to learn some of it, but I had never been pretty exposed to it on an academic sense. And I really liked that the business analytics side of things, leveraging models like machine learning models to generate insight from your data, forecasting your data. So I was able to get an internship like my MBA internship at Microsoft at a team that sits within the finance org, but it is a software engineering slash data science team. So I was able to get in, basically I had the finance background and I just needed to learn the technical skills, the coding. So I did my internship there and I was fortunate to get a return offer after I graduated and I did come back and I’ve been there almost a year and a half now.
Paul Barnhurst:
Great. So thank you for sharing a little bit about that. So I’m, what are some of the differences you’ve seen maybe in finance and just the way things operate, say between Panama and the US right? You’ve worked in different countries, you also studies in Spain, so maybe some of the differences you’ve seen.
Daniel Sousa-Lennox:
Well, even though here in the US at Microsoft, I haven’t technically being in corporate finance, I have partnered a lot with them because they want to make forecasts, they want to use machine learning. So I can speak a bit of it. And I think what I’ve noticed are more similarities than difference actually. And that came as a little bit of a surprise to me because I was expecting, I don’t know, maybe because the difference in country US versus Panama or just sector banking, traditional banking versus tech company. I was thinking that the people in finance were, I don’t know the tools that used were going to be different or the methods methodologies they used or how frequently they adopted new tools. I thought that I was going to find a clear difference there. And it hasn’t been the case so far.
We use the same tools, the models that I used to make at the bank in Panama and the models that I see all the finance teams here doing are still in Excel. It’s still for the most part a manual process. And also there is that maybe a human trait that just is a little bit accentuated in finance, but a little bit of our resistance to adopting new tools or new methods of doing things. And what I mean by that is specifically I work with machine learning and I’m trying to help our finance work transition, their manual TED use long process of forecasting to using ML where it applies or where it can be effective. And there’s some persistence there to making that transition. And that may come from not trusting where the number comes from or the whole black box concept of if you don’t really understand what the model is doing, again leads to the trust issue.
And that’s something that I also saw back in Panama where I used to work. We weren’t working with ML at the time with machine learning, but any new tool that we wanted to bring in that was meant to speak things up, automate, there was a bit of resistance at the beginning and maybe even more so than just the beginning. And that is something that I have noticed here. So I would highlight more the similarities I’ve seen not so much difference right now. I can’t even think of any one difference worth mentioning more so than the similarities.
Paul Barnhurst:
Good. I appreciate that. And at the end of the day, planning is planning, right? I mean, how different can it really be? Yes, there are some clear differences between each company and what FP&A does and responsibilities and things, but that’s good to hear. It’s very similar and I think that’s what I’ve found, whether I talk to different people is it tends to be more similar than different. So I do appreciate your comments and we’ll talk a little bit more about that, about machine learning, the black box, the challenge of change in adoption because we’re definitely in a changing world and it’s coming and being ready for it can be a challenge for some people. So before we jump into that, I would just like to ask what made you decide to focus more on data science and technology within finance? What led to steering that direction after school? Obviously you did an engineering degree, you went straight into finance, and then your role is called software engineer. You moved a little more to the tech side, but working with finance, what led to that? What was the thinking?
Daniel Sousa-Lennox:
Yes, I think a big part of me wanted to be doing something that had a regard a bit more creativeness or a bit more of a creative space. And there is space for great creativity in F P&A and in everything you do. But I felt it was also very easy to get lost in the urgency of how things need to be always do yesterday. And it’s easy to get this autopilot where I need to update this model and I super easy to just not even really think about the numbers you’re inputting, just inputting to get it done as fast as possible. And I found myself falling victim to that a lot.
And I wanted to do something that I had to, again, in everything you can add creativity to it and in everything you definitely think about what you’re doing. But this kind of urgency factor that was always ever present, I was getting a little bit tired of it and I wanted to do something that forced me to be creative. If I have a machine learning task, I need to improve a model, build a model, I cannot shut off my brain and just go through the motion. I have to think I have to take a step back, take a step back and really plan what I want to do and think about what I want to do. So I wanted to move to that more a creative space. But then I also did enjoy my time in finance. I like numbers, I like modeling, I like spreadsheets, I like all of that. So that’s why when the opportunity came of being able to do this specifically for finance people, that was a great deal for me. It was going to be going to building these models, trying to improve these models, but it’s always with the goal in mind of how can I help the people that are doing the job that I used to do make it faster, better, more accurate. So it was like being able to mix both worlds while definitely moving away from that urgency that’s ever present in a budgeting or in a FP&A role.
Paul Barnhurst:
Yeah, I think you summed it up well when you said there’s always urgency, you feel like you’re always behind. It was wanted yesterday and so it limits the time you can spend on coming up with a solution. Thinking creatively, it can be very challenging. I mean obviously we want to do it, but it’s hard. And I can see for you that was you were like, I’m ready for a change. I like some of the work. How can I marry the two without that sense of urgency so to speak?
Daniel Sousa-Lennox:
Yes.
Paul Barnhurst:
Makes sense. Now on the creative side, I will say, I like to joke, what’s the difference between an accountant and an FP&A professional?
Daniel Sousa-Lennox:
What is
Paul Barnhurst:
When an accountant gets creative, they go to jail. When an FP and a professional gets creative, they get promoted.
Daniel Sousa-Lennox:
That’s right. That’s awesome.
Paul Barnhurst:
There you go. That’s my fun. When you said creative, that’s what it made me think of. So next question here. You talked about machine learning a little bit and wanting to do some of those type of things. So let’s talk about Microsoft. How are they using machine learning and AI to help improve the overall forecast to help the FP&A team? So what are some of the things you guys are doing?
Daniel Sousa-Lennox:
Yeah, when I joined, which was right before Chat GPT came out, generative AI came out all first answer without including that. And then I’ll add how has come into the mix. So we’ve been building this standardized framework, which we can talk about that more in detail later. But what we want to be able to do is put machine learning in the hands of finance professionals without them having to go through a data science machine learning course without them having to know the coding Python R or whatever language, having them being able to still take advantage of these models to get highly accurate forecasts at a fraction of the time that it used to be. So how we’re doing it at Microsoft is my team built this tool and we constantly keep adding to it, adding new models, adding new features to make it more accurate or faster. And we also go through the process of evangelizing the tool throughout the organization, throughout the finance organization, which is our main target, and then also with our customers that sometimes they want to hear about what are we doing in finance to incorporate such tools. What we do is basically build the solutions. Then also we teach people how to use it, how to trust or hopefully get them to trust it both internally within the company and externally to some of our big accounts.
Paul Barnhurst:
Got It. Thank you for sharing that. When you talk about the external, that’s how I got to learn about Microsoft and using AI and Mike Tokic which I believe is your manager. He was at AFP last year and he presented with someone else fascinating presentation, talking about these type of things. So I definitely say you guys are doing a good job on the external. That’s how I learned about FINN and the open source there and the different things you’re doing, and I know I’ve shared it with some people before we jump in a little bit more than that and get a little more detail there, I just would love to see what’s an example of how your department has helped Microsoft make a better decision within that process. Maybe some way you’ve supported them, improved a forecast or helped them get insight. So maybe just sharing how having that machine learning and what you’re doing has improved the overall quality?
Daniel Sousa-Lennox:
Yeah, so we have a few teams that have completely adopted the use of machine learning and specifically our tool called FINN, which we’ll talk about in a few minutes, that they have completely adopted this tool and have replaced their current process and what that has meant for them. And one specific teams example is their forecasting process, which they update on a monthly basis. Took them basically about a week, a weeks of one analyst or two analysts process of coming up with an initial number, going to leadership, getting feedback, changing the number, stuff like that. And they were first of all having a bit of trouble keeping track of accuracy, which is something that we’ve noticed across the board. Everything that’s been doing their manual process, they’re forecasting through a manual process, which is every team, they have not been tracking accuracy consistently through each iteration period of updating the forecast.
And the issue with that is, well, it cannot improve, but you don’t measure. And if you have no idea how accurate, it’s because you don’t go back three months later to check what happened and if our predictions were accurate, it’s hard to know if what you’re doing is actually helping at the time it feels like it does, but in the end, are we making the right decisions based on the numbers that we come up with if we’re not going to going back and check. So our tool has helped, for instance, this one team in terms of accuracy to get the revenue that they were trying to forecast from to a 99% accuracy, which is part of it is due to how that business behaves. I cannot say that ML is just magic and it’ll lead to 99% in every single forecast based on how a subscription service behaves.
It’s very well suited for machine learning to come in. So that’s part of it. The other part is our tool, the work it does at testing several different models, testing combinations of several different models. So that has led to them getting ridiculously great amount, number of the accuracy metric, and then the time saving it takes on average for a forecast used run using our tool, it takes about an hour to an hour and a half, which is significantly less than a week, and it’s also an hour and a half where you don’t have to be actively doing anything.
Paul Barnhurst:
Sure
Daniel Sousa-Lennox:
You have a bunch of other stuff to do other than budgeting or forecasting. So it’s an hour and a half where you can turn to whatever it is else that you need to do and then you get your output and then you analyze your output. Now we’re building tools to help you analyze such output. So we’ve seen these across those few teams that have fully transitioned. We’ve seen that increase in accuracy and significantly decrease in time spent to get to a number of such accuracy.
Paul Barnhurst:
Thank you for sharing. That’s a great example and I love how you said machine learning is not the magic bullet, but for certain use cases, many situations it can be highly accurate and then all the time savings. And what it made me think of is, so there’s an organization called FP&A Trends. I had the person who runs it, Larissa on the podcast at one point, they do an annual survey. What I found really interesting is in 2022 they did a survey and they grouped it by how well people thought their forecast or budget was. And so they had 39% said, yeah, my budget is good to great. 16% said it’s basically useless just in general across all boards. But as you went up and looked at those who were using AI and M L, the number went from 39 to 63% that thought their forecast was good or great. And so it just shows using technology with the human and I think you need both can vastly improve the process.
Daniel Sousa-Lennox:
Yes, yes, definitely.
Paul Barnhurst:
It sounds like that’s what you’re seeing.
Daniel Sousa-Lennox:
Yes, yes.
Paul Barnhurst:
That’s great. So next question I want to get into a little bit into FINN and I’m just going to set the stage here. As I mentioned earlier, I saw your boss last year that gave a fascinating presentation about Microsoft and how they were using machine learning. I will say it was my favorite presentation at AFP , really enjoyed it. When you talked about the algorithm you guys were using called FINN, how they had made that open source. Can you tell our audience a little bit about FINN, what it is, how people can use it, maybe talk a little bit about that.
Daniel Sousa-Lennox:
Yes. Yeah. So FINN is a standardized modeling framework we built at our team that looks to allow anyone in finance, actually anyone beyond finance can use it, but we’re targeting specifically our internal finance audience. It allows any of them to produce a machine learning forecast with no need to code anything or to learn what an arena model is and how to code one in Python, none of that. So internally at Microsoft we built a UI, like a web UI where the user can interact with them through dropdown menus. So it’s absolutely a no code tool internally. Externally, like you mentioned, we’ve made it open source source the package which was built in R, which is one of the two most popular languages for ML, probably after Python we built it in R and we made it open source, which means anyone can go into GitHub into the Microsoft prepo and look for FINN, I probably can give you a link later and you can share with the audience and everyone can go and download these our package and run it at their local machine or they can set it up with Azure or your favorite cloud provider and make it run in parallel on the cloud.
All of that can be accessible to you just by downloading our package. So what it does is automates all the tedious processes of doing machine learning, which is you got to prep the data first, make sure it’s how it needs to be for the models to ingest the data. That includes cleaning the data, analyzing if you have outliers and taking care of them. If you do doing feature engineering, which is basically you have a set amount of columns that you have in your dataset and you can add more information to it by decomposing such columns like date for example. You just separate the day from the month. That gives valuable information to models. So FINN automates that part as well. Back testing, which is that process of what’s my model accurate at all, how can I test the numbers? It does that process of benchmarking for you as well.
And then FINN has over 20 different ML models that it tests both individually and as a group. So it does the model selection for you. You don’t have to think of which one was the best. It’ll select automatically which one was the best based on the accuracy metrics. And lastly, it creates your future forecast, how far into the future you want it to be. We can do daily all the way to yearly and everything in between. And all you need to do as a user is bring historical data, high quality as possible, as much historical data as possible, but that’s really the only requirement and Finn will do the rest. And like I said, maybe an hour, an hour and a half, depending on the length of your dataset, you get back your forecast with whichever method you’re trying to forecast.
Paul Barnhurst:
Great. So it sounds like it helps you kind of prep clean up the data in the sense of getting it in the right format for running all the algorithms, running the different methods. Then it compares each of those based on criteria, whether it’s correlation, Z-score, whatever those different statistical means are to look at it and say which of these is best does the backtesting to compare so it can see the variances? And then does it recommend, I’m just curious, does it provide you, here’s the top recommendation, here’s the second, here’s the third, or does it just give you one or what’s that output? I’m curious.
Daniel Sousa-Lennox:
Yeah, the output is it gives you the forecast for every model that FINN has in it, but it checks with a checkbox which one was the best performing one. So you get access to all of them in case if you do want to go deeper and understand what one model does, and maybe for some reason you do want to go a different route, you can do that. You get access to all those results. But it clearly states this model or this combination of models were the ones that led to the highest accuracy in your forecast and therefore these are the future values that we suggest you use.
Paul Barnhurst:
So it sounds like the biggest thing to use it is one, a willingness to try it, trying to figure it out, two data. You got to have enough data to be able to give itSomething to forecast, and you need to have at least a reasonable level of quality. If the data’s really bad, What Doesn’t matter what method you use, you’re not going to necessarily get good results.
Daniel Sousa-Lennox:
Yeah, yeah, exactly.
Paul Barnhurst:
I had that problem once. I did a bunch of statistical analysis when I worked at American Express and just nothing was coming out any good. And so I went and talked to a guy that we had, he was an economics guy, he had a PhD in stats and he looked at all of it. He goes, your data’s just not good enough. You’re never going to get a good answer from this data. And it made me feel better because spent forever on it and I’m like, this is just not very good. I was like, it really taught me the importance of data and if you’re using financial data, it can be a real challenge versus operational data because of accruals and adjustments and all those things that don’t necessarily apply to time in the way operationally it happened, right? They have to be done that way for accounting purposes. And so it reminded the importance of really having operational data when can for a lot of things.
What Would you say about that? Do you think operational tends to be the data you use more than financial to project revenue numbers and those type of things?
Daniel Sousa-Lennox:
I think it’s mostly financial, but right now Finn is able to handle anything that is time series and time series means a number that you want to predict across time. So our use case is finance, finance always, basically. But anything that has a number that you want to predict across time, you can use it. It could be weather if you want it to be. Sure.
Paul Barnhurst:
Got it. That makes sense. So next question here for you. Obviously you work closely with FP&A to improve the forecasting models. So talk to us a little bit about how that process typically goes. You mentioned earlier black box, sometimes not being trust. So maybe just talk about how you work with them and maybe are there any challenges you find or how does that process go?
Daniel Sousa-Lennox:
Yeah, I think the first thing, when the team is ready to try machine learning, the first thing is creating that clarity that, like we said previously, it’s not magic. It’s not going to automatically get whatever it is that you bring in and spin out accuracy because it’s not a crystal ball that looks into the future. So the first thing is the quality that you bring in your data, it’s crucial to as a starting point to even get something decent at first. That’s one. The second one is also creating clarity that it is an e rative process that takes a lot of iterations because at first you get a result, it’s not as accurate as you want. You need to go back. You need to think of what impacts my business that I could add into the data. The other thing that happens a lot is people usually just bring in the historical data of whatever metrics you want to predict, and that’s it.
So you want to predict revenue for your company three months into the future, and all you bring is revenue for the past three years. That could be useful if you have a business that has a high correlation or auto correlation, meaning what happened a year ago significantly impacts what’s going to happen the next year. That usually happens with a subscription business or something. But in general, you need to know what drives your business, what metrics of the economy or what company metrics or industry metrics impact your products. So that’s one thing. Getting the teams to really think through what those are,
If they have them readily available, incorporate that into their data. And if they don’t go through that process of how can I source this data and then join in with my data, because that is going to significantly impact the accuracy of your forecast, especially for certain products and services in certain industries or geographies. So it’s getting that clarity with the teams and also letting them know that once since Finn’s already built, the technical part of it is already done, the hard technical part of it’s already done. So the most important part now is the business expertise that you as a finance person bring of your product, of your service, your domain, and that’s what’s going to make translating that expertise you have into data what’s going to make or break your forecast in general. Because that translates, like I was saying, that translates into adding the appropriate drivers or the appropriate breakdowns of how to make the forecast.
Because you could go as high up or as granular as you want, but maybe there’s a level in between that’s the one that’s going to hit that sweet spot of accuracy. So only you as the finance person or the product owner or the service, you are the one best suited to help translate that knowledge into data. More so that Mike or myself, which we can help with the technical aspects of, yeah, you can toggle this input and maybe change this in when you’re setting up FINN, but 80% of it or more, it’s going to come from what that expertise you have and your ability to translate that into data to be ingested by the models.
Paul Barnhurst:
I really like how you emphasize that. I don’t think that is emphasized enough that you need that person, that expert, that understands the business, that understands those drivers and can bring that judgment to the model to ensure the right data is being ingested at the right level, the right different variables. Hey, if I’m in home building, then I need to understand materials and okay, what’s going on with this? Or that could have a big impact on this expenses that we’re trying to forecast or weather and ice cream sales is always the one you hear, right? What’s going on with that? How would it impact that? So having that understanding is critical. I think it’s really important for people to realize, I think a lot of people fear technology and ai not only for the black box, but also will it take my job? I think sometimes people worry that that’s going to happen. And yes, has technology over the years changed jobs and if some people lost jobs, yes, but the economy changes and adjusts with that. And so I think it’s important to be willing to embrace technology. And speaking of embracing technology, I’d love to just hear a little bit of how generative AI chat GPT everything that’s happened over the last year has changed things for you and how you see that continuing to impact what we’re doing going forward in FP&A.
Daniel Sousa-Lennox:
Yes. I’ll speak to that from two lenses, one of a software engineer since that’s what I do, but then I’ll emphasize more on the FP&A part.
Paul Barnhurst:
Sure.
Daniel Sousa-Lennox:
On the software engineer, which is I’m building these data science tools, these machine learning tools for finance professionals, generative AI has made my ramp up process so much faster, and I think that would apply to even beyond software engineering. But every time I’m dealing with a new problem, it always involves new functions, new packages, new things that I haven’t tried, and being able to ask Chat GPT this is the task I need to do, this is where I want to do it, how would I start? And having speak back, sometimes even the whole answer already,
But even if not the whole thing, just giving you an example that you can test by yourself that’s made the process. I probably was what, six to 10 months before Chat GPT came out. So I already went through that ramp up process without it and then with it, and it’s been significantly better. And then on the FP&A side, and I go back to that kind of urgency that you always have in these types of budgeting or forecasting processes and how you very easily getting this autopilot where we’re just updating the spreadsheet, changing the numbers and not necessarily thinking too much through it. I think generative AI can help, we hope and is helping already with this all this tedious, more autopilot tasks such as updating or getting some insights that then you need to analyze and then you need to see through. So that’s hopefully as it gets more adoption going to reduce the time that you spend on such tasks that in some cases you could literally just shut your brain off and just do it. It’ll take care of all of those and it’ll allow you not to fall prey of those autopilot tasks and then focus more of your time. Because in the end, usually the roles we have in FP&A is financial planning analyst, meaning you analyze not just crunch and input numbers. So hopefully by Gen AI taking over those repetitive tasks, we can embrace more fully the analyst portion of our jobs.
Paul Barnhurst:
I love that. And I’m fully with you. I mean, at the end of the day, I think the way to think about, at least I think of generative AI is a productivity tool like you mentioned, helps improve productivity. It can help with mundane tasks making real quicker. Like coding, I’m sure stuff that may have taken you 10 hours before is now two hours because 80% of it was done by generative ai and you just kind of validate enough to go, okay, this looks right, but oh no, I need to tweak these two or three things and away I go versus, all right, let me go out on Google, find a way to even think about this, spend an hour reading about it, try it, and back and forth, right?
Daniel Sousa-Lennox:
Yes, exactly right.
Paul Barnhurst:
It’s amazing. I know I teach it sometimes to some of my classes and use it and just, it’s amazing to see people’s responses when they realize what it can do. So exciting times for sure. So as we wrap up here, we’re nearing the end of our time together. I’ve really enjoyed this. We have a section that we like to call the get to Know You section. They’re kind of quick questions we ask you at 30 seconds to ask each one of them, kind of some fun questions just so our audience will get to know you a little bit better, Daniel. So the first one is, what is something interesting about you? Something we wouldn’t find online or that not many people know? And you can’t say Texas fan because we know that one.
Daniel Sousa-Lennox:
Okay, yeah. I won’t say Texas, but I will say in the realm of sports, and it’s that I absolutely love sports. I love watching sports, playing sports, and you probably wouldn’t find out about this. I don’t really post stuff on social media, so you’re not going to see me posting that I’m, I dunno, at the stadium watching the Huskies or the Seahawks. But I absolutely love it. I would travel anywhere to watch, especially soccer, football or tennis match. I would go anywhere. My only constraint for why I’m not in every single match out there is finance style constraints and time constraints. Other than that, I have the willingness and the will of going anywhere. Next week I’m driving up to Vancouver in Canada to watch the Labor Cup, which is a tennis tournament. Last week I drove an hour south to play in a tennis tournament. So yeah, that’s my big passion. Sports,
Paul Barnhurst:
It’s obvious. I can tell you’re very passionate about it. And when I mentioned Texas, when we started, I could see the passion about the Alabama game. So I have to ask one question as a follow up. Who’s the greatest tennis player of all time?
Daniel Sousa-Lennox:
Roger Federer.
Paul Barnhurst:
Okay. I was curious to see. You’d say, I know different people have different opinions, so alrighty. So if you could meet one person in the world, dead or alive, who would you meet and why?
Daniel Sousa-Lennox:
I think it would be someone like Leonardo DaVinci or someone from 500 years ago because, and specifically people back then, at least these big names, they were all professions in all professions in one person was, I dunno, he was an engineer, he was a scientist, he was an architect, he was a painter. And obviously they didn’t have as many distractions that we have right now. But I think it would be fascinating to go through how does he and others learn? How do they self teach themselves? Cause they did have people that trained them in the arts or something. But a lot of it, I’m guessing or I know it was self-taught.
And I think that’s something that I’ve been going through in my role now that I’ve been having to teach myself a lot of things in terms of coding, just of machine learning. And I have immense amount of resources. And like I said with Chat GPT, it makes it way easier. So just going through their process of how did they learn only from books and only from talking to each other and to other experts. I think that would be super interesting. And then also just hearing how much you would know about the world or the universe, because even astronomy probably was one of his skills. I think that would be super interesting.
Paul Barnhurst:
Well, I’m going to be a fly on a wall for that meeting. I agree with you. I mean, today we have so many resources at are fingertips to learn and they had a fraction of what we have. So a lot of it had to be self-taught and it would be fascinating to hear them talk about it and how they develop. So I think that’s a great one. So next question, what is the last thing you Googled looked up on YouTube or used generative AI to ask about FP&A finance or Excel?
Daniel Sousa-Lennox:
It wasn’t technically Excel, but I recently built a tool that serves analogous to FINN, but instead of automating machine learning, it automates the exploratory exploratory data analysis, which is really dissecting and understanding your data before you even think of doing ML. So I built that tool and I wanted to add it into the fin workflow and pipeline. And to do that I needed to build an API, which is how can I make FINN call upon this new app that I built without you having to individually run one then the other. So I used all of them, Google, YouTube and Chat GPT on how can I build an API? That was my first time doing that and fortunately I can say that it took some time, but it went successful.
Paul Barnhurst:
I might have to pick your brain. I was recently thinking about a situation where I might need to build an API and I have no clue. So you might get an email from me at some point. So appreciate you sharing that. One last one here. This is one of our favorite, we ask every guest this question have from the beginning, what’s your favorite Excel function or feature? Favorite thing about Excel?
Daniel Sousa-Lennox:
Nowadays, I don’t use Excel as much as I did, but during those five years at the bank, it was my bread and butter every single day. And I think 50% of my time I was either 50% of the time I was doing a VLookup and the other 50% of the time I was doing a pivot table. So one of those two or those two,
Paul Barnhurst:
As soon as you said that, I was like, let me guess, some kind of lookup function. So you’re going to be VLOOKUP or index match, and the pivot table, those two are two of the top ones that people say there’s three are tied, three or four that are tied for number one, I think it’s vlookup, index match, pivot table. And then second is like power query and I think it might be X lookup. Right? So it’s aggregating data and lookups.
Daniel Sousa-Lennox:
Yeah,
Paul Barnhurst:
Those are what finance people need from Excel is the conclusion I’ve come to.
Daniel Sousa-Lennox:
Yes, every day.
Paul Barnhurst:
Yeah, exactly. So we got two questions left here. Last one. If you could offer advice to someone starting a career in FP&A today, if you could give ’em a piece of advice, what would you give them?
Daniel Sousa-Lennox:
It would be to fight that urgency mode that leads you to just going through the motions and updating spreadsheets and just doing the task that at hand without going through that analysis portion. And I know it’s going to be extremely hard because things are due now or even yesterday, but I think that would be my main advice because first, that’s how you’re going to actually grow within your role, your organization in an FP&A role. Because getting those numbers crunched faster, yes, that’s noticeable and that’s impactful and that’s helpful, but in the end, that’s not going to leave the same mark that it would if you have those insights. If you can bring the numbers crunch to your leadership team, but alongside those, you already have insights, you already have ideas, you already have recommendations, that’s how you’re going to stand out, more so than if you just bring really good, nice quick numbers and then they have to do the thinking and then get back to you with more requests. So if you can anticipate those requests and get those insights and bring those up to your team and your leadership team, that’s going to make a significant difference both in your career and how much you get out of it. Also, if you just update spreadsheets all day every three weeks or every end of month close, that would be the advice. But I realize that it’s hard. It’ll be hard, but I think it would be highly, highly worth it.
Paul Barnhurst:
A hundred percent agree. It is hard, but it is worth it. I know when I’ve done it, it’s helped me in my career. I love the couple things you said in there. Anticipate, move away from the urgent and spend time on the insights, find ways to really provide value to the business, not just do your reporting. So very well said. I love that advice there. So last question, if someone wants to get ahold of you, what’s the best way for them to do that?
Daniel Sousa-Lennox:
I think LinkedIn would be the best way. I mean, I’m not as popular as you Paul, so I don’t get that many DMs, so probably a DM. I’ll definitely see it and respond to it. So LinkedIn would be the best way.
Paul Barnhurst:
Great. Yeah, no, I appreciate that. And we’ll make sure to put that in the show notes so anyone can reach out to you if you have a question. Thank you again, Daniel. I’ve thoroughly enjoyed the conversation you’re having on the show. I think people really enjoy learning about some of the things Microsoft’s doing and enjoyed learning about you. So thank you for taking the time to be with us.
Daniel Sousa-Lennox:
Thank you very much, Paul. This was my first time as a guest in the podcast and it was really fun to talk to you and hopefully people find some value in the things that we’ve shared today.
Paul Barnhurst:
I’m sure they will. I know I did, so thank you.